Good, Better, Best – how small steps can drive higher education analytics success
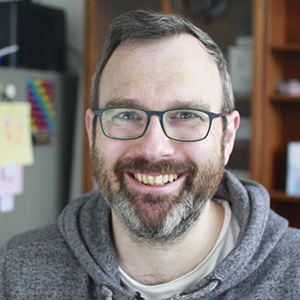
Data analytics offer a way for providers to track and improve key metrics whilst supporting students, but how can they build an analytics culture?
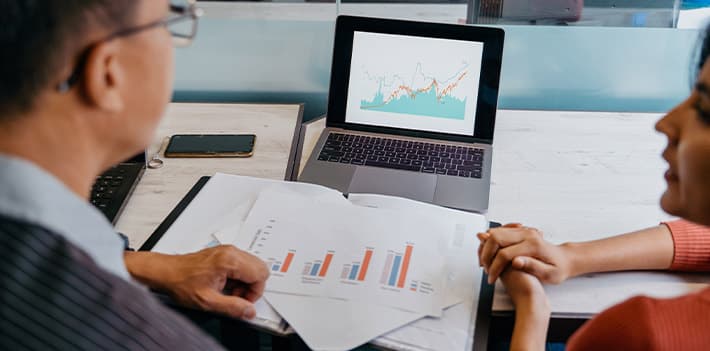
Throughout my career, I’ve seen data analytics help improve student success, progression, retention and wellbeing.
But I have also seen initiatives to deliver analytics in higher education providers (HEPs) stall, fail to deliver actionable insights or fizzle out after initial success.
To utilise analytics successfully, universities must understand how they will use the insights it provides and maintain the infrastructure used to support it.
Steps to success
Analytics is not just about data: any organisation wishing to utilise analytics must set out what it wants to achieve and the methods it plans to use to do it.
This means identifying key metrics to track and then connecting them with target outcomes and planned interventions.
An organisation’s options for intervention are likely to be either text-based nudges or conversations between staff and students. Both are effective, although it has been shown that personal conversations significantly increase the number of students re-engaging with their studies compared to those that just receive a nudge.
Analysis carried out as part of the Onwards from Learning Analytics Project (OFLA) showed that 16% more students re-engaged following speaking to a member of staff versus an emailed nudge.
Once key metrics and targets have been set, HEPs should focus on data. Setting clear guidelines for its collection and use, and building an architecture to support it are vital to success in any analytics project.
Starting the journey
Intervene
Once data teams turn their data into information they will have actionable insights. To find value in these insights, HEPs must focus on the actions the data triggers, and the outcomes of these actions.
You need to identify:
- Who will intervene – which staff member?
- When will they intervene – what is the trigger?
- How will they do it – email, call, automation?
Selecting data
There are lots of data sources for HEPs to choose from, but not all are useful in offering insights. All data should be related to the activity a student has actively engaged in. For example, tracking when a student’s device connects to campus wifi is possible, but this tells you nothing about their activity and engagement.
Interrogating data is also key to success. Staff involved in the selection and processing of source data must ensure they understand the source data and confirm that what the data suggests is the truth. For example, timestamps on device, system and library logins can be wrong – verify they are correct before basing interventions on them.
Student analytics roadmap
As HEPs begin to use data and analytics to instigate interventions with students, their maturity improves.
The journey can broadly be described as moving HEPs from a descriptive model to a prescriptive one; or moving from reporting important activity to predicting behaviours.
This is how we break down each stage on that model:
Good
A HEP’s systems automatically capture student actions in their records; for example, logging into a virtual learning environment, accessing the library or attending a lecture could be recorded.
Bringing this data together can allow a university to know when a student was last active. This is often the first step in identifying issues that are preventing them from engaging in their studies.
Better
At this stage, HEPs can begin to develop more rounded descriptive analytics.
This is also referred to as learning analytics or engagement analytics. The key element here is comparing students to their peers to identify those with relative risk. In the most mature setups, the data a model uses will not rely on manual steps to capture, convert and aggregate it.
HEPs at this stage of maturity use a more sophisticated system than those at the ‘good’ level of development. ‘Better’ systems utilise multiple data sources and provide fewer false positives (or students identified as being at risk incorrectly) while allowing more detailed identification of areas of concern about a student.
This requires a high level of data quality to be maintained so that active students and their cohorts can be identified and compared appropriately.
Best
Predictive analytics is the most sophisticated tool in use currently. Often mislabelled AI, this is typically machine learning where a model is developed that can predict an outcome based on a set of inputs.
It requires a high level of data maturity from multiple data sources, maintained for a period of years. HEP data systems need to provide a history of activity and known outcomes to make predictions.
This allows the model to determine which triggers are most predictive. It consumes significant resources to manage the model and maintain the quality of the data it relies on.
Get started
Regardless of where an organisation starts, students will benefit from supportive interventions to help them succeed. It is important that universities do not let the pursuit of the perfect be the enemy of the good.
It’s OK to be using just one data source. Using just one metric will mean a focus on simple interventions connected to easy-to-identify data triggers.
The first step any organisation can take is to start with the data source they understand best that has the greatest accuracy.
Find out more
About the author
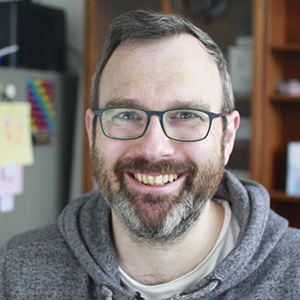
Alongside the other senior analytics consultants, I support the delivery of learning analytics into educational organisations from pre-sales to deployment and maintaining customer relations. I also use my experience of working in student facing services to provide advice on guidance on using analytics for wellbeing.