Value and benefits of text mining
Through text, data mining and analytics we can exploit the vast amounts of information and data generated everyday through economic, academic and social activities.
Executive summary
Businesses use data and text mining to analyse customer and competitor data to improve competitiveness; the pharmaceutical industry mines patents and research articles to improve drug discovery; within academic research, mining and analytics of large datasets are delivering efficiencies and new knowledge in areas as diverse as biological science, particle physics and media and communications.
We have explored the costs, benefits, barriers and risks associated with text mining within UKFHE research using the approach to welfare economics laid out in the UK Treasury best practice guidelines for evaluation.
The global research community generates over 1.5 million new scholarly articles per annum [1]. As the recent Hargreaves report into 'Digital Opportunity: A Review of Intellectual Property and Growth' [2] highlighted, text mining and analytics of this scholarly literature and other digitised text affords a real opportunity to support innovation and the development of new knowledge. However, current UK copyright laws are restricting this use of text mining. To remedy this, Hargreaves proposes an exception to support text mining and analytics for non-commercial research.
In order to be 'mined', text must be accessed, copied, analysed, annotated and related to existing information and understanding. Even if the user has access rights to the material, making annotated copies can be illegal under current copyright law without the permission of the copyright holder.
To date there has been no systematic analysis of the value and benefits of text mining to UK further and higher education (UKFHE), nor of the additional value and benefits that might result from the exceptions to copyright proposed by Hargreaves. Jisc thus commissioned this analysis of 'The Value and Benefits of Text Mining to UK Further and Higher Education'.
We have explored the costs, benefits, barriers and risks associated with text mining within UKFHE research using the approach to welfare economics laid out in the UK Treasury best practice guidelines for evaluation [3]. We gathered our evidence from consultations with key stakeholders and a set of case studies.
There is clear potential for significant productivity gains, with benefit both to the sector and to the wider economy
Key findings
- We found some significant use of text mining in fields such as biomedical sciences and chemistry and some early adoption within the social sciences and humanities. Current UK copyright restrictions, however, mean that most text mining in UKFHE is based on Open Access documents or bespoke arrangements. This means that the availability of material for text mining is limited.
- The costs of text mining relate to access rights to text-minable materials, transaction costs (participation in text mining), entry (setting up text mining), staff and underlying infrastructure. Currently, the most significant costs are transaction costs and entry costs. Given the sophisticated technical nature of text mining, entry costs will by and large remain high.
Current high transaction costs are attributable to the need to negotiate a maze of licensing agreements covering the collections researchers wish to study. - We undertook a number of case studies to explore the economic value and benefits of text mining to UKFHE. Due to the limited uptake of text mining and legal and commercial restrictions, we adopted a stylised approach, focusing on specific small-scale illustrations of the value and benefits of text mining, and the wider potential value and benefits that could be delivered if technical and legal limitations were resolved. Benefits include: increased researcher efficiency; unlocking hidden information and developing new knowledge; exploring new horizons; improved research and evidence base; and improving the research process and quality. Broader economic and societal benefits include cost savings and productivity gains, innovative new service development, new business models and new medical treatments.
- The Hargreaves review suggested that text mining could bring savings and wider innovation potential to UKFHE. The existing legal restrictions on text mining meant that it proved very difficult within the course of this study to source sufficiently robust data to systematically quantify these potential benefits. However, the evidence gathered illustrates that there is clear potential for significant productivity gains, with benefit both to the sector and to the wider economy.
- Legal uncertainty, inaccessible information silos, lack of information and lack of a critical mass are barriers to text mining within UKFHE. While the latter two can be addressed through campaigns to inform and raise awareness, the former two are unlikely to be resolved without changes to the current licensing system and global adoption of interoperability standards.
- Text mining presents an opportunity for the UK, encouraging innovation and growth through leveraging additional value from the public research base. The UK has a number of strengths that put it in a good position to be a key player in text mining development, including good framework conditions for innovation and the natural advantage of its native language. The scholarly publishing market is global, predominantly in English, with global potential for demand for text mining tools and services. This offers opportunities for new service companies as well as current content providers. However, these opportunities are being hindered by a range of economic-related barriers including legal restrictions, high transaction costs and information deficit which is strongly indicative of market failure.
- The technological developments underpinning text mining are relatively recent and hence were not envisaged in previous consideration of the impact of copyright. However, because the process of text mining involves the production and storage of copies of material that may be subject to copyright, there is a new conundrum: the market intervention of copyright – originally intended to protect creative producers – may be inhibiting new knowledge discovery and innovation.
1. Introduction
1.1 Context
Economic, academic and social activities generate ever increasing quantities of data. Businesses collect trillions of bytes of information on customer transactions, suppliers, internal operations and indeed competitors [4]; the global research community generates over 1.5 million new scholarly articles per annum; and social networking sites such as Facebook and twitter enable users to share over 1.3 billion pieces of information/content per day [5].
MGI: creative use of these large data sets in the US health care sector could generate more than $300bn in value per annum
According to the McKinsey Global Institute's (MGI) 'Big Data' report [6], the generation of information and data has become a 'torrent', pouring into all sectors of the global economy and is predicted to increase at a rate of 40% annually. Exploitation of this vast data and information resource can generate significant economic benefits, says the report, including enhancements in productivity and competitiveness, as well as generating additional value for consumers. For example, MGI predict that effective and creative use of these large data sets [7] in the US health care sector could generate more than $300bn in value per annum and reduce national health care expenditures by around 8%.
In the UK also, there is recognition that considerable economic and public value can be generated through better use of our information assets. The Prime Minister recently announced the government's intention to release anonymised NHS records to life sciences companies, in the expectation that research using these data could give earlier access to valuable and innovative treatments for patients, as well as significant, potentially world-leading, innovation, within the UK life sciences industry.
Some organisations, commercial and non-profit-making alike, are already leveraging these vast data and information resources to good effect. For example, a strong element of Tesco's market success has been credited to its mining of customer information from its loyalty scheme [8]. Within the research community, the e-Science and e-Social Science communities are using distributed grid computing as well as text and data mining and analytics to extract new knowledge and hidden insights from large data sets in, for example, the areas of biological science, particle physics and social media. These uses signal the potential of text and data mining [9] to lead to the development of technology businesses [10,11], to increase research productivity and quality [12] and, on a wider social scale, to lead to the discovery of new treatments for serious illnesses such as Alzheimer's [13].
The economic potential is further illustrated by the investment technology giants such as IBM and Microsoft, as well as UK companies such as Autonomy, are making in developing data analytics technologies. Indeed, Gartner Inc. has identified 'Big Data' and 'Next-Generation Analytics' as two of the 'Top 10 Strategic Technologies' for 2012 [14].
However, the full economic and societal potential afforded by this vast sea of information and data is not yet being realised within the UK. Realising the potential requires text and data analytical capability, access to the information and data sources, and involves a range of computerised analytical processes, not all of which are readily permitted within the current UK legislative environment for intellectual property. The latter point was recognised by the Prime Minister in his commissioning of the Hargreaves review, which examined whether the current intellectual property framework is actually hindering innovation and growth in the UK economy.
1.2 Report background
The 2011 Hargreaves report into 'Digital Opportunity: A Review of Intellectual Property and Growth' 15 explored whether the current intellectual property (IP) framework in the UK is hindering innovation and economic growth. In examining the potential obstacles, Hargreaves argued that exception(s) to the existing IP framework are required that: allow shifting between formats; are sufficiently general to enable emerging research tools to be applied; and that cannot be overridden by contracts. Without such exceptions, Hargreaves argues, UK business and research will be unable to reap the full benefits of emerging technologies and business models.
In particular, Hargreaves recommended that:
'Government should firmly resist over regulation of activities which do not prejudice the central objective of copyright, namely the provision of incentives to creators.
Government should deliver copyright exceptions at national level to realise all the opportunities within the EU framework, including format shifting, parody, non-commercial research, and library archiving.
The UK should also promote at EU level an exception to support text and data analytics. The UK should give a lead at EU level to develop a further copyright exception designed to build into the EU framework adaptability to new technologies. This would be designed to allow uses enabled by technology of works in ways which do not directly trade on the underlying creative and expressive purpose of the work. The Government should also legislate to ensure that these and other copyright exceptions are protected from override by contract.' (p8)
The potential of text mining and analytics is highlighted as a case in point by Hargreaves: they afford a real opportunity to support innovation and development of new knowledge although their use in the UK is at present very much restricted by the current copyright laws. (Text mining and text analytics are broadly comparable, the latter being a more recent but roughly comparable term [16]. To aid readability, the term text mining will be used to refer to both in this report.)
Text mining is required if organisations and individuals are to make sense of these vast information and data resources and leverage value. The resources need first to be processed – accessed, analysed, annotated and related to existing information and understanding. The processed data can then be 'mined' to identify patterns and extract valuable information and new knowledge. How these information and data resources are analysed depends on their format. Structured data can be relatively easily 'mined' as the structure can be used to aid processing. Using a computer to automatically analyse information contained in documents is however much more difficult. Most digital documents consist of unstructured text containing flat data, rather than structured and meaningful information, which cannot directly be automatically processed by a computer in a useful way. 'Text mining' therefore involves more complicated processes than structured data mining, and it is the processes involved that give rise to the conflict with copyright law. Given the volume of text generated by business, academic and social activities – in for example competitor reports, research publications or customer opinions on social networking sites – text mining is, however, highly important.
Within UKFHE the potential benefits of text mining have been recognised in several areas. There is limited but significant use of text mining and analytics, especially in biomedical and related sciences (e.g. [17]), chemistry, computing science as well as some exploratory use in the social sciences [18, 19]. Further, UKFHE has been leading the way in developing text mining tools and in the National Centre for Text Mining (NaCTeM) [20] has an internationally recognised centre of excellence. To date, however, there has been no systematic analysis of the value and benefits derived from such usage to UKFHE, nor of the additional value and benefits that might result from the exceptions to copyright proposed by Hargreaves.
Establishing such value and benefits is important not only to UKFHE. The use of text mining in research is likely to aid wider innovation and hence economic and societal benefits, given the central role that the public research base produced by universities plays in innovation [21] and the fact that UK universities generated £59bn for the economy in 2008 [22]. Jisc thus commissioned this 'Analysis of the Value and Benefits of Text Mining and Text Analytics to UK Further and Higher Education' [23].
1.3 Aim, focus and scope of the study
The overarching aim of this study was to explore the value and benefits of the use of text mining and analytics to UKFHE both currently and if Hargreaves exceptions were to be implemented. The research was guided by the following research questions:
- What is the potential for text mining and text analytic technologies and practices in UKFHE?
- What are the costs, benefits (in particular the economic value) and risks of exploiting this potential, for whom, both now and in the foreseeable future?
- What are the main barriers to the exploitation of this potential, and how might they be overcome?
Text mining is an enabling technology with applicability across learning, research and management. The focus of this study is on the public intellectual outputs of further and higher education, rather than (for example) administrative records, and how the application of text mining to these outputs can benefit UK academics, colleges and universities, and thereby the wider UK economy and society. That said, the study draws on the wider use of text mining software in the commercial sector and internationally to inform how it could be applied within UKFHE in the future.
While the focus is on quantitative economic evidence of the value and benefits of text mining, there are significant limitations in the data available; therefore qualitative evidence is used to illustrate key benefits where quantitative data are unavailable.
We originally focused on two key areas highlighted by Hargreaves [24]:
- Where text mining could potentially generate cost savings (and productivity gains)
- Where text mining use in UKFHE could potentially generate wider impact on the economy, for example by leading to wider innovation in products or services
However, during the course of the study, it emerged that the barriers limiting uptake of text mining appeared sufficiently significant to restrict seriously current and future text mining use in UKFHE, irrespective of the degree of potential economic and innovation gains for society. We therefore also explored more fundamental issues relating to economic efficiency and evidence for possible market failure, as well as considering the matter of 'equity' or 'fairness' in relation to the restrictions on text mining. These issues were considered within the well-established framework of welfare economics, adhering to the approach laid out in the UK Treasury 'Green Book' [25]. The study also focused primarily on usage in research rather than teaching. This further narrowed the focus to UK higher rather than further education.
1.4 Study approach
For text mining to be used in UKFHE for competitive advantage (as Hargreaves advocates), there needs to be a better understanding of the value and benefits it can generate, particularly in economic terms. Better evidence is required to help inform the decisions regarding the optimal policy, technical and support infrastructures to help UKFHE exploit the potential that text mining offers. Evidence gathering and analysis needs to be based on methodologically sound techniques that are appropriate to the further and higher education sector. Particular issues for assessment of the value and benefits include:
- Text mining within UKFHE is in relatively early stages of development but generation of benefits can have a long time frame
- Not all economic and social benefits can be captured in financial evaluations but require a broader perspective on economic value and non-market impacts 26
To address these issues we adopted a combination of qualitative and quantitative approaches which drew heavily on the range of cost benefit analyses and evaluation techniques promoted by the UK Treasury Programme Appraisal and Evaluation handbook, the 'Green Book' [27], the principles of which all UK central government departments follow.
The general approach consists of four stages [28].
- Desk research and consultation focused on targeted consultations with key stakeholders to survey current use of text mining in UKFHE and beyond, including: approaches taken; the technical, economic, legal and policy conditions; costs, initial indications of benefits, issues and barriers to uptake
- Baseline evidence was sought in parallel for a range of comparator countries on their economic indicators, policies and practices that could affect their ability to take economic advantage of this emerging technology
- Case studies were originally intended to gather detailed economic evidence of current and innovative practice relating to text mining. However, issues of the legality of text mining, the current limited uptake and commercial confidentiality limited the extent and range of economic data that could be gathered. The study therefore focused on gathering evidence across multiple cases and extant research to produce 'stylised' cases that illustrate key value and benefits – realised or potential – of text mining to UKFHE [29]. These case studies also gathered evidence on where the potential was being limited by the current legal framework as well as technical infrastructure and scholarship systems
- Economic analysis was undertaken using the evidence gathered. This drew on best practice techniques in cost benefit analysis and valuation as recommended by the HM Treasury 'Green' and 'Magenta Books' [30, 31] and best practice in risk assessment as recommended in the HM Treasury 'Orange Book' [32]. The analysis focused on: cost savings (and productivity gains); the potential for generating wider impact and innovation; and the efficiency and fairness of the market
The evidence was gathered and analysis undertaken in accordance with best practice. In particular, it is in line with UK IPO guidelines on good evidence for policy [33]. The figures used in valuations are based, wherever possible, on sector standards and empirical data. However, where such data are unavailable, experts' best estimates are used. Further, given the small scale nature of this study, its limited resources and the difficulties in locating 'real world' quantitative evidence, the economic valuations are indicative rather than general to the whole of UKFHE. However, they provide a reasonable indication of the scale and magnitude of the economic benefits that could be derived.
Additionally, extensive peer review was undertaken to ensure fair feedback and better inform the final report. This was achieved through two means. First, two senior project mentors with additional specialist skills provided internal peer review, regularly reviewing the methodology, evidence and analysis. Second, a peer review workshop was held in February 2012 to analyse the findings and refine the report. This was attended by key text mining stakeholders from a range of areas interested in the value and benefits of text mining in UKFHE and beyond. This also afforded the opportunity to capture stakeholder opinions, which were used to develop short webcasts covering the project findings and the issues involved.
Overall, the approach adopted was influenced by short time scales, the small scale of the project and limited data availability.
As well as presenting the findings of the study, this report also includes four appendices which present the international baseline of text mining and related activities: Appendix A includes an overview of the position on copyright exceptions across a number of developed countries; Appendix B includes a copyright baseline comparisons table and Appendix C includes an innovative country comparison table. A copy of the questions used in the consultation process is provided in Appendix D.
2. Text mining
Text mining is being used in research both within the UK and across the world. As well as NaCTeM, UK institutions using text mining include: University of Manchester, University of Cambridge, University of Oxford, Institute of Education, University of Strathclyde, University of Lancaster, King's College London, University of St Andrews, University of Bangor, London Metropolitan University, University of Surrey and University of Liverpool. Internationally, text mining is being undertaken in, for example, the USA [34, 35, 36], Sweden [37], Japan [38], Australia [39], Israel [40], Germany [41] and China [42].
2.1 Text mining and its rationale
Scholarly journals and data sources are increasingly available in electronic form making them more accessible to researchers and innovators, in theory at least. However, availability does not equate to being able to analyse easily the content to find sought after information or to develop new insights. The reason is two-fold:
- There is too much literature for a researcher to read. The scholarly publication base consists of 11,550 journals, to which 1.5 million articles are added per year [43]. Similarly, text-based research resources such as social networking communications or policy documents are too numerous for a single researcher or group to read.
- While key word searches might reduce the number [44] of documents, there is no guarantee that the search terms have an identical meaning in the documents retrieved. For example, 'tree', 'branch' and 'leaf' have very different meaning in ecology and informatics, something that is easy for a researcher to see but not for a computer.
Text mining offers a solution to these problems, drawing on techniques from information retrieval, natural language processing, information extraction and data mining/knowledge discovery as Figure 1 illustrates.

Figure 1 - Overview of the components of text mining
Text description for figure 1
Stage 1: enhanced information retrieval
Stage 2: linguistic analysis entity recognition
Stage 3: information extraction
Stage 4: data mining/knowledge discovery
Stage 1: enhanced information retrieval
Stage 2: linguistic analysis entity recognition
Stage 3: information extraction
Stage 4: data mining/knowledge discovery
In essence, during enhanced information retrieval (Stage 1), sophisticated keyword searches retrieve potentially relevant electronic documents. The words of the document (and associated meta data) are then processed (Stage 2), using for example lexical analysis (aided by domain-specific dictionaries), into a form that allows a computer to extract structured data (information) from the original unstructured text. Useful information can then be extracted from the documents (Stage 3). For example, chemical names and reactions can be extracted and visualised to enable: increased quality of information through formal semantic verification; increased reader understanding; automated analysis of reaction conditions and results; and building of reusable formal models of chemical reactions [45].
The identified information can then be mined to find new knowledge, meaningful patterns across the retrieved documents (Stage 4) which would be difficult, if not impossible, to identify without the aid of computers. For example, by looking at indirect links in different groupings of bioscience publications, Swanson was able to hypothesise the causes of rare diseases [46, 47].
Exactly how and what can be achieved depends on the licensing, format and location of the text to be mined. Consider the illustration in Figure 2, of a researcher (or developer) who wishes to mine scholarly publications.
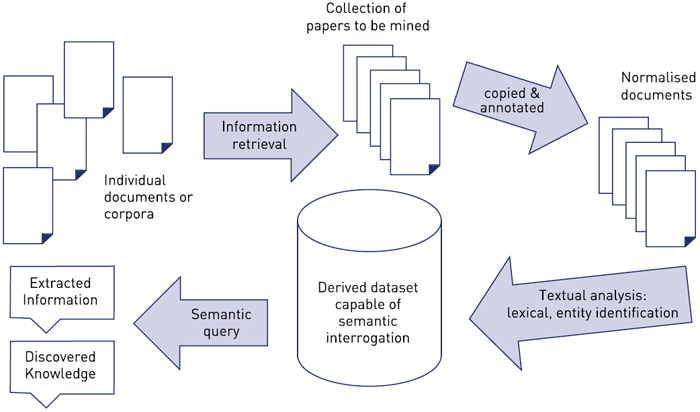
Figure 2 - Schematic overview of the processes involved in text mining of scholarly content
Text description for figure 2
Individual documents or corpora go through information retrieval. This results in a collection of papers to be mined, which are then copied and annotated into normalised documents. The normalised documents undergo textual analysis: lexical and entity identification creating a derived dataset capable of semantic interrogation. Semantic query of the derived dataset results in discovered knowledge and extracted information.
Individual documents or corpora go through information retrieval. This results in a collection of papers to be mined, which are then copied and annotated into normalised documents. The normalised documents undergo textual analysis: lexical and entity identification creating a derived dataset capable of semantic interrogation. Semantic query of the derived dataset results in discovered knowledge and extracted information.
The researcher will have access to various collections (corpora) of abstracts or papers through peer-reviewed publisher journals or through indexing services such as Web of Knowledge [48] or UK PubMed Central [49]. Pre-prints of academic papers on institutional repositories or the web itself provide other sources. Sophisticated information retrieval tools can be applied to the chosen source collections to identify relevant papers to mine for further information. However, before further electronic analysis can begin, the documents to be mined must be 'normalised' i.e. all converted into a similar format to aid processing.
As Figure 2 illustrates, this involves electronic copying of the original documents to produce new normalised and annotated versions. However, as the Hargreaves report highlights, this copying and annotation does not fall under the Fair Dealings exception to UK copyright law [50] and specific permission is required from the copyright holder. This means that to date text mining within UKFHE focuses on corpora of Open Access [51] documents included in collections such as UK PubMed Central [52] or where researchers have negotiated permission through personal contacts with specialised publishers. The processes illustrated in Figure 2 apply equally to text mining of research resources such as digitised literature, business reports or social networking communications. The original research material must first be copied and normalised and therefore appropriate permission is required.
Text mining has applications in all parts of the research process
2.2 Applications of text mining in UKFHE and beyond
Text mining has applications in all parts of the research process from literature review and hypothesising, through experimentation and analysis to generalisation, peer review and publishing. Our investigation revealed six broad categories of use – systematic review of literature, developing new hypotheses, testing hypotheses, building reusable representations of knowledge, improving the quality of text-based artefacts and improving usability of research literature. This list is, however, not exhaustive.
- In systematic reviews of literature, text mining is used to automatically identify literature that should be reviewed by researchers wishing to establish the current state of knowledge in a particular field. The mining takes place across both traditional peer-reviewed academic journals and grey literature such as technical reports, policy documents and pre-prints. Researchers can use the information extracted to identify relevant documents from a much wider source pool, including from other disciplines and non-traditional sources. This enables efficiencies. For example, Thomas and O'Mara-Eves showed that text mining enabled identification of the relevant works with only 25% of the manual effort otherwise needed [53]
- To develop new hypotheses, articles from often disparate topics are text mined to identify interesting intermediate topics and linkages. These intermediate linkages can be used to generate hypotheses which can be tested through investigation. For example, in the biomedical sciences, Swanson used approaches similar to text mining to hypothesise how pre-existing drugs could be used to target different diseases ranging from Raynaud's Disease [54] to Alzheimer's Disease [55]. These hypotheses have been subsequently validated experimentally [56]
- Testing of hypotheses can be achieved by mining collections of documents to see if their content confirms or refutes a hypothesis. For example in the humanities, recent text mining of digitised correspondence from the enlightenment period [57] brought into question the commonly held assumption that the French enlightenment had been heavily influenced by England
- Reusable representations – models or concept maps which present distilled knowledge in a concise and reusable form – can also be generated through text mining. In the biomedical sciences, these representations are in the form of biological pathways within cells [58]. Exploration of these pathways allows better understanding of biological systems and analysis of genomic data [59]
- The quality of documentation can be assessed through text mining. For example, by comparing linguistic structures the readability of a document can be assessed [60]; and by also examining recognised conceptual structures the content can be compared to expected standards. This can enable, for example, assessment of the quality of educational materials in teaching, user manuals or public engagement [61]
- The usability of the research base can be enhanced through text mining to extract metadata automatically. The efficiency of searching is enhanced when 'marked-up' documents, annotated with metadata, are made available to other researchers. Further, the vast amount of text produced on websites, blogs and social media such as twitter can also be investigated using text mining where copyright holders allow, providing a highly important, rich research resource for understanding the economic, social and environmental contexts in which we live. For example, the recent analysis of messages exchanged on twitter during the English riots of 2011 showed that twitter was 'not to blame for inciting riots' [62].
In the disciplinary areas where text mining has been adopted, there are many different examples of domain-specific applications of the broad categories of use described above. For example, Rodriguez-Esteban [63] provides a summary of applications of text mining within the biosciences.
Businesses text mine their documents and public blogs, twitter feeds and web sites for business intelligence
Within business, text mining is used for roughly comparable tasks. Businesses text mine their documents and public blogs, twitter feeds and web sites for business intelligence – identifying emerging trends, exploring consumer preferences and competitor developments. They use the information extracted to competitive advantage, improving and producing new products and services. Text mining is particularly used in larger companies as part of their customer relationship management strategy and in the pharmaceutical industry as part of their research and development strategy. For example, the pharmaceutical industry text mines patents and scholarly literature in order to uncover potential new drug targets, or to identify alternative uses for existing drugs [64].
New start-ups such as ScrapperWiki (which extracts useful text and data from web pages, pdfs and spreadsheets) are beginning to emerge where text mining is a core part of their business. In more established organisations, such as Autonomy and IBM, text mining and analytics are becoming increasingly important revenue streams. Text mining is also used in legal and security fields. For example, the Ministry of Justice in Korea has been exploring the establishment of an 'Intelligent Legislation Support System' developing text mining for review of legal cases and precedents [65]. And within the security field it is used significantly in anti-spam measures [66] and has been explored at least in the context of counter terrorism [67]. Further, there is growing interest within law enforcement for automated document classification; this goes beyond traditional subject-specific classifications to determining similarities in authorship, common themes, intentions, etc.
3. Costs, benefits, barriers and risks
We explored how text mining is being used, the associated costs, benefits and the barriers, risks and other issues during 17 interviews with a range of researchers, tools and service providers, and representatives from business and non-commercial organisations. All have a strong interest in the value and benefits of text mining within UKFHE.
The following themes emerged:
- Costs include access, transaction, entry, staff and infrastructure costs
- Benefits include: efficiency; unlocking hidden information and developing new knowledge; exploring new horizons; improved research and evidence base; and improving the research process and quality
- Broader economic and societal benefits were also highlighted, such as cost savings and productivity gains, innovative new service development, new business models and new medical treatments
- Barriers and risks. In general those consulted felt that there were significant barriers to uptake of text mining in UKFHE. These include: legal uncertainty, orphaned works and attribution requirements; entry costs; 'noise' in results; document formats; information silos and corpora specific solutions; lack of transparency; lack of support, infrastructure and technical knowledge; and lack of critical mass
These broad themes (presented in no particular order) and observations are discussed in further detail below [68].
3.1 Costs associated with text mining
3.1.1 Access costs
Where text mining explores copyrighted materials, the copyright holders may require extra payment to allow their material to be used in text mining. This is in addition to the purchase of the right to view the materials. Indeed in some cases the user (or more likely their institution) may need to pay four different costs to enable the materials to be text mined – traditional access (reading) costs, the right to copy, the right to digitise and then the right to text mine. As several of those consulted highlighted, this means that most text mining is limited to exploring Open Access documents where no additional charges are incurred.
In some cases the user (or more likely their institution) may need to pay four different costs to enable the materials to be text mined
3.1.2. Transactions costs
Transaction costs in this context relate to the effort required to enable text mining to take place. This is principally associated with obtaining permission to mine particular corpora of documents. As several of those consulted noted, the nature of publishers' contracts means that it is often ambiguous regarding whether text mining is permissible; not being specifically excluded from an agreement does not imply permission, and it can take significant effort to find the correct contact and then a definitive response. Where additional permission (and payment) is required, this may further prolong the discussion. For example, establishing permission to digitise alone takes roughly the equivalent of 1 FTE [69] as part of the national SHERPA/RoMEO service which offers information about publishers' policies with respect to self-archiving pre-print and post-print research papers [70] – transaction costs associated with mining copyrighted material may be considerably more.
Such transaction costs mean that text mining in UKFHE is mostly limited to Open Access sources, abstracts or full texts or where the individual researcher/group already has a well-established relationship with publishers. This was, for example, the case in 'Digging into The Enlightenment: Mapping the Republic of Letters' [71], where a corpus of 53,000 18th-century letters was text mined.
3.1.3. Entry costs
Entry costs refer to the resources required to develop and/or configure text mining tools to be used within a specific context. There are some generic tools available that require little configuration; however, higher end tools generally require adaption and significant training before they can be used in a different domain. For example, if a researcher wishes to use one of NaCTeM's higher level tools, they generally first need to explore with NaCTeM what the specific requirements are. NaCTeM then undertakes the required developments. Once the refined tool is available, it must be 'trained' to understand the key concepts and relationship with the domain by a domain expert.
Such entry costs are generally built in to research funding proposals; however funding is not always successful and lack of understanding of the importance of text mining and the need for such high entry costs can lead to comments such as 'the world doesn't need another text mining project' [72].
3.1.4. Staff costs
Text mining is currently a very specialised activity, requiring significant technological and analytical skills as well as domain expertise. As more than one of those consulted observed, not only may there be a significant cost associated with training and development of the required skills, but as the demand for text mining expands it may become more difficult and costly to retain experienced text miners. This is true both within UKFHE and within wider business. For example, the 'Big Data' report [73] forecasts a shortage of 140,000–190,000 people in the USA with the necessary deep analytical skills to develop and support data and text mining.
3.1.5. Infrastructure costs
Text mining over large collections [74] requires significant storage and computational resources. For example, as discussed in 2.1, copies of all the documents need to be made and annotated, and large data repositories built.
3.2 Benefits and opportunities
3.2.1. Efficiency
A key benefit of text mining is that it enables much more efficient analysis of extant knowledge. The ability to extract information automatically cuts down the time spent on ensuring coverage of domain knowledge in the literature review process. For example, given the sheer volume of scholarly publications now available in the biomedical fields, it could take a human researcher several years to analyse the corpus to identify all relevant sources for a particular problem. Using text mining to identify relevant material could drastically cut down the time required. (See the case studies of section 4 for some examples.) Further, if the text mined documents were annotated with the semantic information that has been extracted and were then made available for reuse, key resources would be found more quickly.
The unlocked information can lead to new knowledge and improved understanding. For example, text mining has been used to identify new therapeutic uses for thalidomide.
This efficiency saving is equally applicable to the vast range of electronic research sources used in research. For example, the Institute of Education's UK Educational Evidence Portal (eep) [75] enables researchers (and lay people) to find evidence from 33 reputable UK sources (with over 500,000 documents) through a single searchable point of access. Its aim is to radically change the practices of educational researchers by significantly reducing the time they spend searching for appropriate evidence. It does this in two ways. First, it enables efficiencies by eliminating the need to search multiple websites individually. Second, a proto-type web interface enables researchers to identify relevant information more quickly from the lists of information returned by the searches.
This proto-type service was developed as part of the Jisc-funded ASSERT and ASSIST projects and integrates a number of text mining tools and methods into the eep, including automatic classification of documents, automatic clustering of search results by similar document content, and automatic identification and highlighting of key terms within documents [76]. Using such text mining-enhanced services reduces the manual effort required from researchers to undertake a systematic review by 75% [77].
A commercial example illustrates another potential efficiency that could accrue in UKFHE: analyses of business sectors undertaken using text mining of the web cost one tenth of similar analyses undertaken by traditional consultancy companies. If one considers that large commercial organisations can spend hundreds of thousands of pound on sectoral analysis, this is a significant efficiency and cost saving.
All these efficiencies can increase productivity. More detailed examples of efficiency savings that could be accrued in UKFHE are illustrated in the case studies, section 4.
3.2.2. Unlocking 'hidden' information and developing new knowledge
The enormous volume of academic publications and grey literature means that there may be underlying connections between different subtopics that could not be found without automated analysis. The potential links found between diseases and drugs developed for other purposes mentioned in section 2 are a good example of the unlocking of this hidden information. The unlocked information can lead to new knowledge and improved understanding. For example, text mining has been used to identify new therapeutic uses for thalidomide [78].
3.2.3. Exploring new horizons
In some areas text mining is transforming not just how research is done but also what is researched; new horizons and research questions are being explored. For example, a whole new area of digital humanities has emerged. Research in this area is not only leading to better understanding of the information and social-cultural significance embedded in historical artefacts; it is also providing enhanced tools and methodologies to improve understanding of the multi-media world in which we now live.
3.2.4. Improved research and evidence base
Semantically-annotated corpora or reusable representations and domain dictionaries provide a significantly enhanced research resource when made directly available to other researchers and developers. This access could be through Open Access agreements or through contracts with copyright holders that allow text mining and comparison with other corpora. The key benefit here is access to the derived knowledge of others in a form that can be easily interrogated and reused. This is not just a question of efficiency but also of availability. The eep portal [79] of educational evidence and the ChEBI dictionary of molecular entities (Chemical Entities of Biological Interest) [80] (discussed in case study 4.4) are good examples of such improvements in the research and evidence base.
Nano-publishing also has the potential to improve the research and evidence base. It is based on small publishable pieces of information, such as an assertion about something that can be uniquely identified and attributed to its author [81]. Individual nano-publications expose individual assertions and could be cited in scholarly articles. Text mining of such assertions could help track and verify the development of ideas and chains of logic. They could also be used to monitor the impact of particular assertions.
3.2.5. Improving research process and quality
The availability of both text mining tools and the reusable semantic outputs (annotated corpora or knowledge representations) is helping to improve the research process itself as they provide new tools and methods that can be applied in innovative ways. Not only do they enable new horizons to be explored but these tools can also be used to help triangulate findings. For example, a researcher can use text mining to check that their traditional literature review has covered the relevant domain of knowledge. As one researcher reported, this method identified a subset of documents that he had not examined in his traditional literature search. This was because the subset of documents came from a different sub-discipline where they used different terminology for a key concept.
The automated text mining tool had identified this synonym through its analysis despite the fact that it had not been directly learned during the initial tool training. Similarly the building of reusable representations, such as genomic pathways, enables new analyses to be undertaken. Finally, there is interesting work being developed that may allow researchers to trace the origin and development of scientific findings within academic publications. This would allow automatic identification in breaks in the scientific logic, where work had been questioned or retracted, and more accurate identification of the basis of claims. Such a system would aid the quality of research.
3.2.6. Broader benefits
The broader economic benefits identified by those consulted include: cost savings and productivity gains; innovative new service development; and new business models.
Cost savings and productivity gains from using text mining to explore the scientific research base or consumer data are already in evidence. For example, within the pharmaceutical industry collaborative ventures between traditional competitors explore the existing knowledge base to reduce the costs of drug discovery. In some pharmaceutical companies as much as 40% of their R & D is collaborative. Innovative new services, based in part at least on text mining, are beginning to emerge such as SciVerse Applications [82] which is being developed by Elsevier in collaboration with NaCTeM.
New business models may develop for existing business. For example, some copyright holders are exploring allowing their content to be mined for free as 'hits' will attract more visits to their costed service. This is similar to the successful model where some publishers allow Mendeley [83] to provide free excerpts of their content as this can lead researchers to the full documents on their websites, which must then be purchased. Further, as more than one of those consulted highlighted, the business models that emerge may transform the technology/internet business space – similar to the recent transformation brought about by 3G mobile technologies.
The potential for societal benefits are significant, particularly with regards to finding drug treatments or cures for serious diseases such as Alzheimer's and diabetes. However, given the long period of drug trials, establishing a precise value for such ongoing research is problematic. The 'Digging into Data Challenge' [84] project 'Data Mining with Criminal Intent' [85], developed data mining tools that explored the evolution of legal proceedings at the Old Bailey, providing new insights into the development of plea bargaining, and rising rates of convictions. Additionally, some of the visualisation techniques developed to help researchers analyse documents have the potential to better convey research findings and other complex ideas to general audiences.
Environmental benefits have still to be investigated in detail. Research in related areas such as information management [86] and cloud computing [87] suggest the potential for energy savings where duplicate copies of resources can be eliminated. However, as text mining involves making copies and annotating vast amounts of documents, this actually suggests an increased environmental impact would result, as additional storage disks and servers are required. A key issue for further investigation would be the difference in environmental impact of multiple corpora-specific text mining solutions and, say, a central text mining repository for UKFHE.
3.3 Barriers, risks and issues
3.3.1 Legal uncertainty, orphaned works and attribution requirements
As the Hargreaves report points out, at one level the legal position is quite clear – permission from the copyright holder is required before the digital copying and annotation required as part of text mining can be undertaken. However, where institutions already have existing contracts to access particular academic publications, it is often unclear whether text mining is a permissible use. The resource implications of seeking clarification can be significant.
It is often unclear whether text mining is a permissible use
The situation is further complicated where there are orphaned works, where the rights holder is unknown or cannot be contacted. Further, as the law currently stands, copyright law can be overwritten by contract law. So even if, as Hargreaves suggests, there were to be an exception that allows text mining of copyrighted materials for non-commercial research, there could still be considerable restrictions, leading to uncertainty. In the opinion of more than one of those consulted, this uncertainty was a key reason for limited uptake of text mining in UKFHE to date.
The risks to an institution associated with unapproved text mining are significant. One example was given where a single researcher had undertaken some text mining activity on an experimental basis without realising it may not be permitted. This single incident caused all institutional access to a complete set of journals being suspended by the content provider for a week (even though it was ambiguous whether contractually text mining was permissible or not). Such penalties can have severe implications for the ongoing business of a university.
Even where text mining is allowed within publisher contracts, licensing terms that require the full attribution of derivative works developed in the text mining process can effectively prevent text mining usage. For example, the Open Access publisher BioMed 88 has such a licence, allowing text mining and the production of derivative works, provided the relevant attribution is made. However, where text mining is used to identify new knowledge derived from cross-article analysis of patterns, it is effectively impossible to identify all relevant attributions that contributed to the new derived knowledge [89]. This therefore means that such text mining cannot be undertaken.
3.3.2 Entry costs
The entry costs associated with development and 'training' of text mining tools for use within a different topic from that for which they were originally designed were also identified as a significant barrier to uptake of text mining. Investment in training for researchers is also required. Significant tools have been developed through various initiatives in, for example, biomedicine and chemistry. However, there is little uptake in other disciplines, which a number of those consulted felt was at least in part due to such entry costs. The Digging into Data Challenge [90] is however beginning to support and encourage development within the humanities.
3.3.3 Noise in text mining results
Text mining of documents may produce errors. False connections may be identified or others missed. In most contexts, where the noise (error rate) is sufficiently low, the advantages of automation outweigh the possibility of a higher error than that produced by a human reader. However, in some contexts even low error rates cannot be tolerated. While this can be viewed as a barrier, text mining is still used in a range of safety critical areas such as drug development. In such cases the extraction of information is only partially automated, with a (human) domain expert checking the automated selections. More extensive (and complementary) mining of the full text could also reduce error rates, where the full text is available
The tendency to store papers lodged within institutional repositories as pdfs only further contributes to the problem
3.3.4 Document formats
The format of many documents also limits the amount of text that can be mined. This is particularly an issue when the documents are stored as images or 'pdfs', as it is difficult to identify and extract relevant metadata. There is no standard fully automated way to convert such documents into more text mining-friendly formats. Further, where these more friendly formats are available, publishers may impose additional charges for access. The tendency to store papers lodged within institutional repositories as pdfs only further contributes to the problem. XML is the preferred format for text mining.
3.3.5 Information silos and corpora specific solutions
Corpora of documents or individual orphaned documents [91] for which text mining agreements have not been made must be excluded, leading to inaccessible silos of information and limiting the effectiveness of text mining. Some copyright holders allow text mining of their corpora only through bespoke text mining services. While this does enable the corpora to be mined, it is in isolation from other potentially highly relevant documents. This type of solution still leads to unconnected information silos.
3.3.6 Lack of transparency
For many, text mining is perceived as a black box where corpora of text documents are input and new knowledge is output. Where researchers do not have the technical knowledge or skills to understand the internal workings of text mining, or do not have access to the corpora or text mining tools, text mining is effectively opaque. This lack of transparency limits use in three ways. First, it discourages researchers from using what they do not fully understand. Second, without good understanding of the process involved, the potential of new and innovative applications may be missed. Third, if the process and research data are not transparent then it is impossible for others to reproduce the results – a critical requirement if proposed new knowledge is to be accepted by the academic community.
For example, it will be impossible for other researchers to reproduce the results of text mining a corpus of journal articles or digital documents if they do not have access to all the documents in the original corpora. For one of our participants, there was a real risk that such lack of transparency might severely limit his ability to get his work published in peer-reviewed journals. Given the requirements of the national Research Excellence Framework [92], which assesses the quality of research in UKHE, this has the potential to impact negatively both on an institution's standing and the individual researcher's career.
For small research groups... lack of a central infrastructure... may rule out use of text mining.
3.3.7 Lack of support, infrastructure and technical knowledge
Text mining is a highly specialised activity, which creates additional annotated copies of corpora and large information repositories. For small research groups or individual researchers, lack of a central infrastructure to support this may rule out use of text mining. Participants in non-scientific areas also felt that it was difficult to obtain funding for technical infrastructure and support. Some of those consulted also felt that the current level of mathematical understanding with respect to certain application areas was also limiting what could be achieved. Further, in many areas, the domain specific dictionaries used by text mining tools do not yet adequately cover the range of terms and concepts used, nor the rich, formal linguistic information on behaviour of words required. These are expensive to build and maintain, although this process is easier where full text sources can be used to construct the dictionaries.
The concern was also expressed that opening up access to text mining could negatively impact the infrastructure and hence the quality of services that publishers provide.
However:
"As an OA publisher we are certainly happy for people to use our published content for text mining purposes. As you mentioned, there is some concern about the load that this may place on our web servers, so we are in the process of setting up an FTP site where researchers will be able to download the XMLs of all of our published articles for text mining purposes, which should help reduce the load on our servers.
"Practically speaking, the impact of text mining on our servers has not yet become a problem, and I'm sure that the load from search engine spiders is a lot higher than from researchers trying to text mine our content. Also, given that we host all of published articles using Amazon's Simple Storage Service (S3) my guess is that it would take quite a few researchers doing text mining on our content at the same time to cause any real problems."
Paul Peters, Head of Business Development, Hindawi Publishing Corporation
And:
"We haven't had any problem with server load performance from robots text mining the journal sites. Previously, there were occasions that site performance would degrade from what appeared to be out-of-control scripts hitting a single article. In that case, we would block the IP of the script. But since we implemented the new software, we haven't seen this problem come up.
"There are no restrictions for text mining our content other than respecting the crawl-delay in robots.txt (currently set to 30 seconds) and fetching content from one journal at a time"
Public Library of Science (PLoS) who, among other things, run the largest journal in the world, PLoS ONE
3.3.8. Lack of critical mass
As identified by several of those consulted, there is a lack of critical mass of text mining in many disciplines in UKFHE. Lack of discipline-specific exemplars or buzz, along with the preceding barriers, may be limiting uptake. The preceding barriers highlight some key risks in undertaking text mining: breaking the law, inability to publish, incomplete coverage, drain on time and 'noise' in results.
A major pharmaceutical company used text mining tools to evaluate 50,000 patents in 18 months [93]. This would have taken 50 person years to achieve
Finally, as pointed out by some of those consulted, there are also risks associated with not utilising text mining. These were categorised as the potential for: financial loss; prestige loss; opportunity loss and brain drain. Further, there are some tasks that simply could not be achieved without using text mining. For example, a major pharmaceutical company used text mining tools to evaluate 50,000 patents in 18 months [94]. This would have taken 50 person years to achieve manually, meaning that it would not even have been contemplated. Also, the Evidence for Policy and Practice Information and Co-ordinating Centre at the Institute of Education reported, when commenting on using NaCTeM text mining technologies to undertake a systematic literature review: 'It was only possible to conduct a review with such a broad scope in such a comparatively short space of time by using the new technologies of automated text mining.' [95]
4. Case studies of the economic value
We undertook text mining case studies to collect, where possible, direct evidence across the whole value chain of the costs and benefits of text mining and text analytics which would enable generalisations pertinent for UK HE/FE to be drawn.
Sourcing suitable case studies to cover the range of potential uses and fields proved problematic for reasons mentioned earlier: text mining is used in just a few specialised fields; where text mining is taking place, data on its use and value are sparse and often anecdotal; legal and commercial restrictions limited participation. The five case studies presented in this section were therefore selected pragmatically; they focus on specific small-scale examples of the value and benefits of text mining and the wider potential value and benefits that could be delivered if technical and legal limitations were resolved.
The case studies were in the main undertaken by telephone, skype and email; however, in order to widen coverage desk research of extant material was also undertaken. For the protocol design, we drew on the findings of the initial consultation, Yin [96] and cost and benefits analysis techniques employed by the team in e.g. BIILS (The Benefits of ICT Investment Landscape Study) [97].
4.1 Text mining to support literature review in systems biology
Researchers in the biomedical sciences trying to develop new understanding and medicines to treat diseases are increasingly struggling to keep up to date with relevant literature. PubMed alone has 21 million citations for abstracts or full articles and this is increasing at a rate of two per minute [98]. This case study is based on the literature review and synthesis undertaken by Professor Douglas Kell in 2008–2009 to produce the highly cited journal article – 'Iron behaving badly: inappropriate iron chelation as a major contributor to the aetiology of vascular and other progressive inflammatory and degenerative diseases' [99]. It provides insight into the benefits of and barriers to text mining, illustrating how the full potential value that text mining could offer is yet to be realised.
Kell's research started from a chance discussion with another academic when visiting the USA which led him to wonder about the role iron might play in a number of diseases. Rather than starting with a specific hypothesis, Kell decided to explore the literature to see what conclusions might reasonably be drawn regarding the role of iron in a variety of diseases. He felt it important to explore literature across as many relevant domains as possible as biomedical literature can be extremely segmented with researchers and medical practitioners tending only to be interested in research within their own narrow specialist field, such as cardiovascular disease or ophthalmology. This means that important cross links could be missed.
Kell used tools such as Kleio [100], a knowledge-enriched information retrieval system for biology, and Facta [101] which finds associated concepts using text analysis. He also undertook searches using Web of Knowledge [102], Scopus [103] and Google Scholar [104]. He was able to identify 2,469 articles to cite in his paper which concluded that the role of excess iron had been underappreciated, and that in combination with certain chemicals its activity underpins a great many physiological processes that degrade over time. Kell identified two benefits of text mining: being able to find a larger amount of relevant documents and being able to cover multiple fields. As Box 1 below illustrates, increased (multi-disciplinary) coverage was achieved without the overheads of involving a multi-disciplinary team.
Box 1 - Efficiency savings associated with using text mining to support information retrieval
A useful indication of research coverage is number of sources – distinct journals – that an article cites. In 2007, within the biological sciences in the UK, the average number of sources per article was 3.80, with an average of 91.50 sources per 1,000 references [105]. Kell's 2009 article contained 942 distinct sources in 2,469 references.
- Sources per 1,000 references in Kell's paper = 381.53
- Factor by which coverage was increased =
- Sources per 1,000 refs in Kell' s paper / Average sources per 1,000 refs = 4.17
- This gives an 'increased coverage' factor of 4.17.
Further, this increase in coverage has the added advantage that it is identifying more links between articles – it identifies a network of interrelated facts (and articles). So the amount of useful information is more than simply the 2,000 or so references relating to iron [106]. It also relates to the number of links between the papers referenced and hence is enabling a greater depth of knowledge.
A useful indication of research coverage is number of sources – distinct journals – that an article cites. In 2007, within the biological sciences in the UK, the average number of sources per article was 3.80, with an average of 91.50 sources per 1,000 references [105]. Kell's 2009 article contained 942 distinct sources in 2,469 references.
- Sources per 1,000 references in Kell's paper = 381.53
- Factor by which coverage was increased =
- Sources per 1,000 refs in Kell' s paper / Average sources per 1,000 refs = 4.17
- This gives an 'increased coverage' factor of 4.17.
Further, this increase in coverage has the added advantage that it is identifying more links between articles – it identifies a network of interrelated facts (and articles). So the amount of useful information is more than simply the 2,000 or so references relating to iron [106]. It also relates to the number of links between the papers referenced and hence is enabling a greater depth of knowledge.
The research took around 50 weeks, 10 hours per week. As Kell noted , if text mining could have been used to automatically summarise the papers [107], he could have saved considerable time. While automatic summarising is technically possible, the current copyright law prevents this from being implemented except in individual corpora that contain Open Access to full texts.
Box 2 below illustrates the efficiency saving through automated summarising that could accrue if Hargreaves exception were to be implemented. While this is not a direct comparison (as reading a summary is not the same as reading a full text), as Tenopir et al's longitudinal studies [108] have illustrated researchers are having to change their reading approaches to find better ways of keeping up to date with the ever increasing body of scholarly literature.
Box 2 - Potential efficiency saving that automated summarising could deliver
- Time taken to read paper in order to summarise contents – 31 minutes [109]
- Time taken to read an automated summary – 5 minutes,
- Time saved through automated summarising = 26 minutes
- Assuming average academic salary of £48,000 and 1,650 working hours per annum, then:
- Cost saving per summary = £12.61
According to Tenopir et al [110] the average academic in the sciences reads on average 204 unique articles per year. Assuming the same reading behaviour across all disciplines, automated summarising through text mining could therefore lead to cost savings per academic per year equivalent to £2,572. With over 144,000 academic staff in UK HE this would imply possible research efficiency savings of over £370m p.a.
- Time taken to read paper in order to summarise contents – 31 minutes [109]
- Time taken to read an automated summary – 5 minutes,
- Time saved through automated summarising = 26 minutes
- Assuming average academic salary of £48,000 and 1,650 working hours per annum, then:
- Cost saving per summary = £12.61
According to Tenopir et al [110] the average academic in the sciences reads on average 204 unique articles per year. Assuming the same reading behaviour across all disciplines, automated summarising through text mining could therefore lead to cost savings per academic per year equivalent to £2,572. With over 144,000 academic staff in UK HE this would imply possible research efficiency savings of over £370m p.a.
In summary, this case study of using text mining in the literature review process highlights the additional coverage that can be achieved through text mining, indicating how this value might be assessed. It also highlights that significant additional efficiencies could be achieved if there was text mining-access to full journal articles with standardised metadata.
4.2 Using text mining to expedite research
Text mining potentially offers two ways of decreasing the expensive and lengthy drug discovery life cycle. Internally, the pharmaceutical industry uses text mining to help identify information required to develop new drugs as well as to explore new application areas for existing drugs. This involves targeted information retrieval, entity extraction and finding links and associations across documents. As this is a highly competitive area, commercial considerations mean that it is not possible to make public the efficiency gains achieved; however, the extent of text mining undertaken by the pharmaceutical industry indicates that it finds the process valuable [111, 112].
The pharmaceutical industry is also using text mining with external partners to explore 'big' problems which they would not otherwise have the resources to do. New start-ups such as ConnectedDiscovery [113] bring together interested pharmaceutical companies and researchers to provide knowledge management solutions for pre-competitive pharmaceutical research. Additionally, projects such as SESL [114] are exploring the development of brokering services that use semantic technologies incorporating text mining to push appropriate information from a range of sources to researchers in response to a single query, thus saving researchers' time. However, these approaches are not without problems. For example, SESL could only use Open Access materials and indeed has been unable to move from a proof of concept to a working business model.
Box 3 below from the Wellcome Trust illustrates some of the problems.
Box 3 - Transaction costs associated with text mining across disparate corpora
In the free-to-access, UKPMC repository [115] there are 2930 full-text articles, published since 2000, which have the word 'malaria' in the title [116].
Of these 1,818 (62%) are Open Access and thus suitable for text mining without having to seek permission [117]. However, the remaining 1,112 articles (38%) are not open access, and thus permission from the rights-holder to text-mine this content must be sought.
The 1,112 articles were published in 187 different journals, published by 75 publishers.
As publisher details are not held in the UKPMC database, the permission-seeking researcher will need to make contact with every journal. Using a highly conservative estimate of one hour research per journal title [118] (ie to find contact address, indicate which articles they wish to text-mine, send letters, follow-up non-responses, and record permissions etc) this exercise will take 187 hours. Assuming that the researcher was newly qualified, earning around £30,000 pa, this single exercise would incur a cost of £3,399 [119].
In reality however, a researcher would not limit his/her text mining analysis to articles which contained a relevant keyword in the title. Thus, if we expand this case study to find any full-text research article in UKPMC which mentions malaria (and published since 2000) the cohort increases from 2,930 to 15,757 [120].
Of these, some 7,759 articles (49%), published in 1,024 journals, were not Open Access. Consequently, in this example, a researcher would need to contact 1,024 journals at a transaction cost (in terms of time spent) of £18,630; 62.1% of a working year.
In the free-to-access, UKPMC repository [115] there are 2930 full-text articles, published since 2000, which have the word 'malaria' in the title [116].
Of these 1,818 (62%) are Open Access and thus suitable for text mining without having to seek permission [117]. However, the remaining 1,112 articles (38%) are not open access, and thus permission from the rights-holder to text-mine this content must be sought.
The 1,112 articles were published in 187 different journals, published by 75 publishers.
As publisher details are not held in the UKPMC database, the permission-seeking researcher will need to make contact with every journal. Using a highly conservative estimate of one hour research per journal title [118] (ie to find contact address, indicate which articles they wish to text-mine, send letters, follow-up non-responses, and record permissions etc) this exercise will take 187 hours. Assuming that the researcher was newly qualified, earning around £30,000 pa, this single exercise would incur a cost of £3,399 [119].
In reality however, a researcher would not limit his/her text mining analysis to articles which contained a relevant keyword in the title. Thus, if we expand this case study to find any full-text research article in UKPMC which mentions malaria (and published since 2000) the cohort increases from 2,930 to 15,757 [120].
Of these, some 7,759 articles (49%), published in 1,024 journals, were not Open Access. Consequently, in this example, a researcher would need to contact 1,024 journals at a transaction cost (in terms of time spent) of £18,630; 62.1% of a working year.
In summary, this stylised case study illustrates that the use of text mining can expedite research, but that high transaction costs can effectively inhibit its use.
4.3 Using text mining to increase accessibility and relevance of scholarly content
As this case study of the Jisc JournalArchives [121] illustrates, text mining can be used to provide more efficient searching, which returns higher quality results than traditional information retrieval techniques. Jisc JournalArchives contains a selection of journal archives that have been licensed for perpetual access by member institutions. MIMAS has recently developed a service that enables simple and fast conceptual searching across more than 450 journals published by Brill, Institution of Civil Engineers, Institute of Physics, ProQuest, Oxford University Press and the Royal Society of Chemistry. The aim of this subscription service [122] is to enable researchers to access well-targeted content through three simple clicks from one central interface rather than having to visit multiple content providers' websites and negotiate their differing interfaces. As Box 4 below illustrates, it increases researcher efficiency.
Box 4 - Resources savings accrued through using Jisc JournalArchives
Increased accessibility and relevance in information retrieval
Searching on Jisc JournalArchives for journal articles relating to 'graphene' returned 137 results with one click. Each of the individual papers can then be accessed through two further clicks – the second to select the paper from the return list and the third to download the pdf. At a conservative estimate, this takes less than 45 seconds, assuming sufficient internet bandwidth.
Carrying out the same search manually over the individual archives would involve at least five clicks per archive – visiting the archive, logging in, searching for graphene, selecting the journal article to read and downloading the pdf.
Further, the returned list is automatically ranked for relevance. So at a conservative estimate, the researcher can select papers to read in less than one minute. Using Tenopir and King [123] figures that the average US academic spends 5.2 minutes selecting a paper when browsing collections,
- Time saved through text mining enhanced paper selection = 5.2-1 = 4.2 minutes
- Assuming median researcher salary of £48,000 and 1,650 working hours per annum, then:
- Cost saving per paper selected = £2.04
This illustrates a very real productivity gain – the researcher only spends approximately 1/5th of the time they would normally spend on paper selection.
Tenopir et al [124] estimate that an average academic selects 204 papers per year. This implies a cost saving of £416.16 per academic per year. Applied across the UK HE sector this would indicate £59.9m worth of academic time could be saved through the streamlined search process [125].
Increased accessibility and relevance in information retrieval
Searching on Jisc JournalArchives for journal articles relating to 'graphene' returned 137 results with one click. Each of the individual papers can then be accessed through two further clicks – the second to select the paper from the return list and the third to download the pdf. At a conservative estimate, this takes less than 45 seconds, assuming sufficient internet bandwidth.
Carrying out the same search manually over the individual archives would involve at least five clicks per archive – visiting the archive, logging in, searching for graphene, selecting the journal article to read and downloading the pdf.
Further, the returned list is automatically ranked for relevance. So at a conservative estimate, the researcher can select papers to read in less than one minute. Using Tenopir and King [123] figures that the average US academic spends 5.2 minutes selecting a paper when browsing collections,
- Time saved through text mining enhanced paper selection = 5.2-1 = 4.2 minutes
- Assuming median researcher salary of £48,000 and 1,650 working hours per annum, then:
- Cost saving per paper selected = £2.04
This illustrates a very real productivity gain – the researcher only spends approximately 1/5th of the time they would normally spend on paper selection.
Tenopir et al [124] estimate that an average academic selects 204 papers per year. This implies a cost saving of £416.16 per academic per year. Applied across the UK HE sector this would indicate £59.9m worth of academic time could be saved through the streamlined search process [125].
The Jisc JournalArchives facility also increases the quality of the journal articles selected for further investigation through three means: it ranks the articles identified based on semantic content analysis; it provides a summary of the content; and it provides a list of other articles which are contextually similar. This includes identification of previously unknown links between documents.
Anecdotal evidence from users suggests that lists of conceptually similar articles are particularly helpful in improving the quality of literature reviews; however, current legal/process restrictions limit the value that can be achieved. For example, the Autonomy IDOL software automatically summarises the documents in the collections as part of the initial indexing process. However, the agreements with the rights holders prevent display of this derivative product. As in the systems biology case study (4.1), were this facility available it could increase research productivity by a factor of 6.2 126.
In summary, this case study illustrates the value of efficiency savings that text mining can make, as well as benefits of improved quality of literature reviews.
4.4 Reusable models and curation
Models of metabolic, genomic and chemical pathways and molecules integrate knowledge about processes and/or structures into a coherent system, which can then be accessed and reused. Within systems biology, pathway models are expressed in SBML [127] and are stored in a database. They not only provide graphical representation which aid visualisation, but also form structured databases of biological knowledge, which can be updated as scientific understanding evolves [128]. Examples of pathway databases include iPath, BioCyc or KEGG Pathways [129].
These reusable models are developed from existing knowledge primarily that stored in scholarly articles. Creation of such models is however time consuming if undertaken manually. Typically the first stage is to curate the relevant information contained within the scholarly articles into a relational database. This curation is resource intensive, often leading to significant bottlenecks. For example, ChEBI [130], which is the only free information source on small molecules of biomedical interest, is estimated to be growing at a rate of 100,000 items per annum (200% increase p.a.). ChEBI already has a large backlog of items to be curated and struggles to train/employ sufficient curators [131]. Using text mining to speed up the curation process is the only realistic way to reduce backlog and keep up with data flow [132]. For example, Alex, Grover et al [133] showed that curation time could be increased up by 1/3 when text mining is employed along with subjective feedback from a human curator [134]. Box 5 below illustrates the type of cost savings associated with assisted curation.
Box 5 - Staff time and cost savings associated with assisted curation
In Alex, Grover et al's [135] experiment, curators were asked to curate documents relating to protein–protein interactions (ppi) in biomedical literature. Relevant papers were first identified and normalised using text mining techniques. Name and entity recognition was also undertaken using natural language processing. The normalised paper and automatically-identified ppi information were then fed in to an in-house editing and verification tool. The curator then read through the electronic papers selected to identify relevant information for curation, supported by the ppi information already automatically identified. This significantly helped the curator identify and select appropriate information for curation. Text mining-assisted curation required 1/3 less time than manual curation.
Based on Alex, Grover et al's [136] figures for curation of four papers:
- Curator assisted, text mining supported [137] - 170 records with 3.42 minutes average time per record
- Manual - 121 records with 5.20 minutes average time per record
[138]
- Time saved per record = 5.20 − 3.42 = 1.78 mins
- Assuming an average pay for a curator of £36,040
- and 1,650 working days pa
- Cost saving per record = £0.65
So if context relevant assisted curation were employed in a database with 100,000 new entries pa
- Potential annual cost savings = £65,000
Given that curator costs for manual curation of 100,000 entries would be £189,301, 'assisted curation' represents a 34% saving in curator costs
This nominal saving allows significantly more information to be extracted, making 'assisted curation' more productive.
- Productivity = Assisted curation records extracted per minute / Manual records extracted per minute = 150% (gain of 50%)
In Alex, Grover et al's [135] experiment, curators were asked to curate documents relating to protein–protein interactions (ppi) in biomedical literature. Relevant papers were first identified and normalised using text mining techniques. Name and entity recognition was also undertaken using natural language processing. The normalised paper and automatically-identified ppi information were then fed in to an in-house editing and verification tool. The curator then read through the electronic papers selected to identify relevant information for curation, supported by the ppi information already automatically identified. This significantly helped the curator identify and select appropriate information for curation. Text mining-assisted curation required 1/3 less time than manual curation.
Based on Alex, Grover et al's [136] figures for curation of four papers:
- Curator assisted, text mining supported [137] - 170 records with 3.42 minutes average time per record
- Manual - 121 records with 5.20 minutes average time per record
[138]
- Time saved per record = 5.20 − 3.42 = 1.78 mins
- Assuming an average pay for a curator of £36,040
- and 1,650 working days pa
- Cost saving per record = £0.65
So if context relevant assisted curation were employed in a database with 100,000 new entries pa
- Potential annual cost savings = £65,000
Given that curator costs for manual curation of 100,000 entries would be £189,301, 'assisted curation' represents a 34% saving in curator costs
This nominal saving allows significantly more information to be extracted, making 'assisted curation' more productive.
- Productivity = Assisted curation records extracted per minute / Manual records extracted per minute = 150% (gain of 50%)
In summary, this stylised case study of using text mining to aid curation and development of reusable representations illustrates the potential cost savings that could be accrued and that additional information can be identified to improve the reusable models. Overall this exemplar suggests productivity gains equivalent to increasing the curation output by 50%.
4.5 New services and business models
Mendeley [139,140], an award winning company formed in 2008, enables researchers to discover, manage and share scholarly communications. Currently it has over 1.5 million users, with around 3.6 million unique visits per month. Interestingly, while the founding directors are all German, they chose to base the company in London because: over 90% of scholarly publications are in English; the head offices of a number of major publishing companies are based in the city; there is a significant cluster of world-leading research centres near London; and the UK is one of the best markets for venture capital.
At its core Mendeley consists of a large catalogue of references, built from the references uploaded by its users and augmented with two page abstracts from a number of publishers [141]. Users access their personal reference store and the wider catalogue through either a web interface or desktop application. A key feature is that the Mendeley system tags the references with anonymised social usage information.
Mendeley's business model is based on providing valuable services to their various stakeholder groups in and around the scholarly communication management platform that they have designed. Researchers are provided with four valuable features: (i) the ability to better organise their research papers and references; (ii) enhanced discovery through their large catalogue of papers, through social discovery and through automated algorithmic discovery; (iii) facilities to collaborate on papers through shared repositories; and (iv) automatic backup of their papers and reference lists. As Box 6 below illustrates, these facilities help improve researcher efficiency.
Box 6 - How Mendeley improves research efficiency
Mendeley automatically extracts citation details from textual analysis of uploaded documents and abstracts. While researchers still need to check the accuracy of the extracted details, this automatic extraction significantly decreases the time required. Anecdotally, researchers report that setting up a reference library of hundreds of documents in Mendeley takes minutes, compared with days to enter this information manually or cobble it together from separate databases.
The 130,000 collaborative groups on Mendeley strongly suggest, as does the quote below, that researchers find the collaboration tools highly valuable.
"I work with a group of researchers in my topic area online and with Mendeley we are able to share resources quickly and hold group discussions with participants from all over the world."
Jamie Bogle, Mayo Clinic, Research Fellow, Medicine
Mendeley automatically extracts citation details from textual analysis of uploaded documents and abstracts. While researchers still need to check the accuracy of the extracted details, this automatic extraction significantly decreases the time required. Anecdotally, researchers report that setting up a reference library of hundreds of documents in Mendeley takes minutes, compared with days to enter this information manually or cobble it together from separate databases.
The 130,000 collaborative groups on Mendeley strongly suggest, as does the quote below, that researchers find the collaboration tools highly valuable.
"I work with a group of researchers in my topic area online and with Mendeley we are able to share resources quickly and hold group discussions with participants from all over the world."
Jamie Bogle, Mayo Clinic, Research Fellow, Medicine
Publishers are another significant stakeholder group for Mendeley. In addition to publishers' interest in the referencing statistics of the papers in their corpora, several have been working with Mendeley to establish a new business model. Three major publishers [142] have signed agreements to provide Mendeley with two introductory pages per paper within their collections. Mendeley indexes these along with the reference and abstract in the Mendeley catalogue. When a researcher searches for one of these papers, Mendeley returns the two introductory pages and a link to the relevant publisher's website. This significantly increases the 'click-through' rate to the publisher's content. The publishers' willingness to work with Mendeley on this feature illustrates the potential business advantages.
Mendeley also provides enhanced article/journal usage statistics for institutional libraries through their new 'Mendeley Institutional Edition' co-developed with SWETS [143]. This provides enhanced access information, which can help institutions better to evaluate the impact of their research. The usage statistics also enable libraries to see how much value their institution gets out of a subscription contract. There is also an active third party developer community. Currently, 1,009 API keys have been registered, although it is estimated that the full developer community is two to three times larger. Mendeley sees an active third party developer community as a key way of delivering innovative services to its user base. It actively encourages its growth through, for example, its recent 'Binary Battle Apps for Science Contest' [144], which attracted 40 entries.
Mendeley views development of the database and API platform as of core value to the business and its future direction, assigning eight staff, 25% of the whole workforce to these tasks. Currently, text mining in Mendeley focuses on helping researchers with information retrieval and automated extraction of referencing details, providing enhanced metadata which helps them manage and make (social) linkages across papers more efficiently. The business and academic communities have expressed interest in more extensive text mining facilities. Mendeley is investigating whether this can be developed and rolled out in light of the current legal framework.
"We see enormous commercial potential in text mining, and especially in allowing third-party developers to build text mining tools on top of Mendeley's infrastructure and data. Due to the uncertainties with UK legislation, we are currently exploring opportunities for setting up text mining projects through our US subsidiary, on US-based cloud computing infrastructure. However, since most of our team and infrastructure is based in the UK, this introduces delays and overhead cost, and will potentially lead to Mendeley creating future jobs in the US rather than the UK."
Dr Victor Henning, CEO & Co-founder, Mendeley
In summary, this case study illustrates how new business models could evolve to deliver innovative services and value to researchers and institutions. The types of service possible are limited by the current copyright legislation, but the potential for new services is clear. Given the predominance of English as the language of research and business, the highly active technology and multi-media sector and the availability of venture capital funding, the UK is well placed to be at the forefront of such developments, provided the legal frameworks are conducive to such activities.
4.6 Reflections on the case studies of the value and benefits of text mining
The preceding stylised case studies further highlight that, while text mining can lead to efficiency gains as well as valuable new knowledge and resources, there are significant barriers that are preventing realisation of the full potential. High transaction costs and the lack of ability to text mine over the full range of scholarly publications were identified as particularly significant barriers.
The data that could be gathered were extremely limited both due to commercial confidentiality and also the limited use of text mining. That said, the case study participants all expressed a strong wish that they could take much wider advantage of text mining's potential.
5. Economic analysis of the value and benefits
Improved understanding of how text mining in UKFHE can generate wider economic benefit is an important part of the evidence base underpinning discussions about text mining and whether the Hargreaves-recommended legislative change is necessary. The Hargreaves report [145] highlighted two core areas of potential economic and social benefit and value:
- Where text mining could potentially generate cost savings and productivity gains
- Where text mining in UKFHE could lead to wider innovation in products or services with broader economic and social benefit
We examined both of these areas and also went further to examine the implications of current barriers to text mining.
The current copyright law-driven restrictions on text mining, particularly the text mining of scholarly journals, appear to be inhibiting its wider use or take-up in UKFHE. This situation applies irrespective of the magnitude of any wider economic or social gains that text mining could generate. This observation raises some fundamental questions about the nature and structure of the market for text mining of scholarly journals. These include whether there is evidence for market failure (which would be detrimental to the economy and society overall) as well as the issue of 'equity' or fairness in current market operations – are copyright-driven barriers to text mining in UKFHE preventing society from deriving a fair share of the return on public investment in research?
Therefore in addition to the first two areas of discussion (the potential of text mining to generate cost-savings and its wider innovation potential), we also consider the issues of possible market failure and equity. We finish with reflections and conclusions on the economic potential of text mining to UKFHE.
5.1 Cost savings and productivity gains
Although most text mining activity in UKFHE research has been in specialist areas such as biomedical sciences and computer science [146], there appears clear potential for use in every branch of university research. Different disciplines may use different terminologies and 'ontologies' and require tools tailored to their subject 'dictionaries'. However, all disciplines share the basic principle of requiring systematic reviews of literature (which is essentially search for 'prior art') – and this is time consuming and resource intensive. There are a number of potential process benefits from text mining:
- Time saving: doing a task such as literature review in less time that it would otherwise have taken
- Improved quality and robustness of conclusions: increase coverage of material
- Increased output: for example, more research papers
- Process innovation: enabling a task that would otherwise be impossible.
The existing legal restrictions on text mining meant that it proved very difficult within the course of this study to source sufficiently robust data to enable quantification of the benefits arising. Many of the identified exemplars of text mining and related activity were either heavily circumscribed by issues of confidentiality or remained too small scale to be amenable to quantitative analysis to elicit 'hard' value.
However, we have presented a small number of case studies and given some indication of the types of savings and process improvements to be made. For example:
- 'Text Mining to Support Literature Review in Systems Biology' illustrates how text mining can support time savings and productivity through enabling additional coverage with increased quality of output, with an 'increased coverage factor' of 4.17
- 'Text Mining to Improve Accessibility of Scholarly Content' also shows how text mining can increase research efficiency by performing a filtering process which helps target research efforts better, saving and making better use of time
- 'Reusable Models and Curation' shows how text mining-assisted curation can support as much as a 50% increase in curator output
Some other examples from a range of disciplines can be found in a number of published papers on text mining such as 'Seeding the survey and analysis of research literature with text mining' [147] and 'What the papers say: text mining for genomics and systems biology' [148].
If text mining enabled just a 2% increase in productivity corresponding to only 45 minutes per academic per working week, this would imply over 4.7 million working hours and additional productivity worth between £123.5m and £156.8m in working time per year.
However, while data limitations meant that it was not possible to undertake detailed modelling of value generation, an indication can be given of the likely scale and significance of the value that could be generated through text mining. Taking the literature search capability alone, text mining has the potential to make a very real difference to quality and coverage of the search, being able to target, screen and filter material and hence both directly save time and enable better use of the time saved. The prospective gains could be substantial.
This can be observed in the context of the size and scale of the UK research base itself and the related magnitude of efficiencies that the text mining innovation would bring. UKFHE is a considerable UK industry sector and productivity gains in UKFHE are significant for the wider economy. In 2008, UK HE alone (ie not including FE) directly employed more than 372,000 people and generated over £33bn of GDP [149, 150].
There are currently over 144,000 full time equivalent academic professionals (teaching and research) working in UK higher education [151, 152]. Using data from the Higher Education Statistics Agency (HESA) for UK academic salaries, the median salary for a UK academic falls into a band of between £42k and £55k, which translates to between £26 and £33 per working hour [153]. If text mining enabled just a 2% increase in productivity – corresponding to only 45 minutes per academic per working week [154] (and looking at CIBER's analysis of the impact of eJournals [155], this is very much an underestimate), this would imply over 4.7 million working hours and additional productivity worth between £123.5m and £156.8m in working time per year.
The potential for productivity increases of this magnitude are clearly significant. The resource and value implications should also be seen in the light of how substantial an impact they could have if text mining became embedded in research practice and part of the overall value chain of the research and scholarly communications publishing system.
A 2008 study by Cambridge Economic Policy Associates (CEPA) and commissioned by the UK Research Information Network 156 undertook detailed analysis of the resource flows within the 'scholarly communications system' across the world and in the UK [157]. The 'scholarly communications system' was defined as encompassing:
'the combination of the publishing and distribution of peer-reviewed articles in scholarly journals, and the provision of access to such journals by publishers, academic and non-academic libraries, and other channels'. [158 159]
The study assessed all of the activities, costs (cash and non-cash) and funding flows associated with the scholarly communications base to make an estimate of the system-wide costs of the full research value chain.
Scholarly publishing is a global industry and at a global level the system-wide costs were estimated to be in the region of £175bn. However the most revealing aspect of the CEPA study was not so much the total estimated economic value of the scholarly publishing communications system but in where the study found the majority of the resource costs were incurred. These comprised £115.8bn for research production (the underlying research and article preparation), £6.4bn for publication and distribution, £2.1bn in providing access to the articles (library costs) £50.3bn in user search and print cost and user reading of the articles. Figure 3 presents an overview of the resource costs (cash and non-cash) and funding flows in the global communications system, showing the concentration of resource costs across the different parts of the value chain.
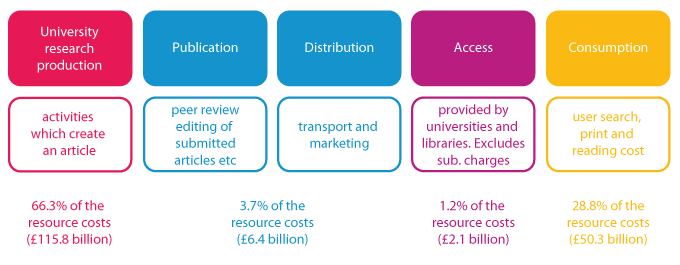
Figure 3: Overview of the resource costs (cash and non-cash) in the global communications system
Text description for figure 3
Overview of the resource costs (cash and non-cash) in the global communications system
University research production (activities which create an article) - 66% of the resource costs (£115.8 billion)
Publication (peer review editing of submitted articles etc) and distribution (transport and marketing) - 3.7% of the resource costs (£6.4 billion)
Access (provided by universities and libraries, excluding sub. charges) - 1.2% of the resource costs (2/1 billion)
Consumption (user search, print and reading cost) - 28.8% of the resource costs (£50.3 billion)
Overview of the resource costs (cash and non-cash) in the global communications system
University research production (activities which create an article) - 66% of the resource costs (£115.8 billion)
Publication (peer review editing of submitted articles etc) and distribution (transport and marketing) - 3.7% of the resource costs (£6.4 billion)
Access (provided by universities and libraries, excluding sub. charges) - 1.2% of the resource costs (2/1 billion)
Consumption (user search, print and reading cost) - 28.8% of the resource costs (£50.3 billion)
The report concluded that the most resource-intensive elements of the system were concentrated, not in the publication stages, but in the original research production, in peer review and in the costs incurred in access and in the consumption and use of the research articles. This was true of the UK as well as at a global level. Therefore innovations such as text mining that help increase researcher efficiency in research production, search and reading articles would have a substantial positive effect.
'Researchers incur much larger costs in terms of searching for, printing and reading journal articles... The biggest scope for cost savings is by increasing researchers' efficiency in searching for, browsing, downloading, copying and reading journal articles...' [160]
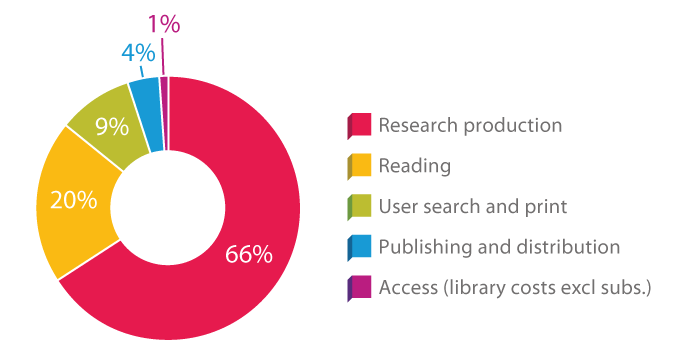
Figure 4: Overview of the resource costs (cash and non-cash) in the global communications system
Text description for figure 4
Overview of the resource costs (cash and non-cash) in the global communications system
- 66% research production
- 20% reading
- 9% user search and print
- 4% publishing and distribution
- 1% access (library costs excluding subs.)
Overview of the resource costs (cash and non-cash) in the global communications system
- 66% research production
- 20% reading
- 9% user search and print
- 4% publishing and distribution
- 1% access (library costs excluding subs.)
The balance of the resource costs within the communications system is also shown in Figure 4.
There can be little doubt that academic researchers would embrace text mining if it became a more accessible and permissible technique. The modelling work undertaken by CEPA uses studies undertaken in the USA on the reading behaviour of academic staff [161]. These studies showed considerably more articles being read after the availability of electronic journals than before, from 150 journal articles per year in 1977 to 280 [162] per year in 2005, with an average time taken of 140 hours per year. Tenopir et al comment:
'...results show that university faculty on average read more in not much more time; have increased the variety of methods used to identify needed articles; rely more on library provided articles; read for many purposes, finding journal articles valuable for these purposes; and, because they make choices based on what helps them to get their work done, will readily adapt to new technologies that are convenient to their information seeking, reading and work pattern' [163]
These changes were in reaction to the advent of electronic journals with technology enabling improved access to material, rather than reflecting text mining usage directly. However the evidence on academic behavioural change in response to opportunities to access content shows a state of 'readiness' for technology such as text mining.
There can be little doubt that academic researchers would embrace text mining if it became a more accessible and permissible technique
The current limitations on text mining have precluded in-depth modelled analysis of the value of specific examples of text mining; however, putting text mining capabilities into the context of the UKFHE research base gives an indication that the scale and magnitude of the value that could be created would be significant.
Furthermore, analysis of potential productivity gains in higher education does not take into account the deeper value that may be generated by any discoveries, or new research insights that could contribute to new innovation. We discuss these additional benefits below.
5.2 Wider impact through innovation
Text mining has considerable potential to 'unlock' knowledge and help leverage maximum value from the higher education research base, at a time when maximising such value is seen as a high policy priority.
The newly published Government strategy towards innovation, 'Innovation and Research Strategy for Growth' [164 ], which proposes a raft of measures to open up access to data and information to stimulate innovation, is strongly underpinned by economic evidence and analysis, summarised in a BIS economics report [165]. This report draws on current analytical thinking to present innovation as underpinning the productivity gains that drive economic growth and social welfare.
The potential of text mining in UKFHE for supporting wider innovation in the economy is even greater
It concludes that while the UK has a number of distinctive strengths, it is not currently one of the leading global innovation nations, being outranked overall by a number of others including the USA, Japan, Germany and Sweden. Other Scandinavian countries, including Denmark and Finland, also score more highly than the UK according to the European Innovation Scoreboard [166]. Improving the UK's position is a key aim of the new innovation strategy.
Text mining in UKFHE is in itself an innovative process, which has the potential to deliver significant productivity gains to UKFHE, most notably in the conduct of research. However, the potential of text mining in UKFHE for supporting wider innovation in the economy is even greater.
Text mining is not a 'stand alone' technique but is potentially a core part of the research discovery process; when considering how text mining could generate value or support innovation it needs to be viewed:
(a) in the context of the research base in which it could be embedded and
(b) in the potential for new cutting edge services and business models that could be in demand globally and could drive business to the UK
5.2.1 The research base
Higher education and the public research base are recognised as having key roles in the innovation process alongside a wide range of influences and supporting factors such as training, skills and intellectual property, as well as governance regimes, manufacturing base, enterprise access to finance etc. Extensive research by NESTA has recognised that a strong research base is one of the six wider framework conditions necessary to foster innovation [167, 168]. There is substantial public investment in the research base every year (in 2010 68% of the £6.9bn research investment in UK higher education institutions was publicly funded) [169]. Maximising the knowledge to be extracted and diffused from that research base is seen as a high priority for innovation policy [170]. UK research is regarded as being high quality, 'with more articles per researcher, more citations per researcher, and more usage per article than researchers in the USA, China, Japan and Germany' [171].
Maximising the knowledge to be extracted and diffused from [the UK] research base is seen as a high priority for innovation policy
A concerted, extensive, interdisciplinary effort is increasingly seen as vital for society to be able to successfully meet the future challenges for sustainable patterns of living, including adapting to demographic change and sustainable use of natural resources [172]. Text mining can help facilitate this, being able to harvest knowledge from across disciplines. The need for more cross-disciplinary and collaborative effort is part of the drive towards 'open innovation' [173], which text mining can support.
A recent article by Porter on 'Mining external R&D' [174] sees text mining as central to open innovation. He highlights 'the desirability of companies' awareness and intellectual interchange concerning externally conducted research'; no company can rely on their own research alone, they need to know what is happening elsewhere. An interest in 'pre-competitive' research is becoming more widespread (with the pharmaceutical industry for example) as well as the essential search for 'prior art', which is at the basis of patent applications. Porter also shows that a significant degree of 'cross-disciplinary knowledge transfer' can be involved in research, citing the example of a single paper in 'Materials Science' referencing papers published in 17 different subject categories, 'Who can keep up with research advances by reading the literature over such a span?' [175]. The techniques and processes of text mining offer a way forward.
The BIS economics paper [176] draws attention to two broad types of innovation that can drive growth: radical innovation and incremental innovation. The first 'radical' type represents the 'breakthrough' that makes a fundamental change, such as the development of the internal combustion engine, which had a fundamental impact on transport. The second 'incremental' innovation is the improvement of a process or concept, such as increasing the engine's fuel efficiency.
The process of discovery enabled by text mining could also be seen in these terms. At one level there may be the 'golden nugget' to be found; the path-breaking new idea that could lead to new inventions and radical innovation. Text mining holds the tantalising possibility that from among the 1.5 million journal articles published every year, the golden nugget is waiting to be found such as a breakthrough in Alzheimer's treatment or similar discovery that would profoundly impact on society and health.
From a more prosaic, but no less important, perspective there is potential for text mining to support incremental innovation by seeking and finding patterns across large volumes of material to shed new insights into existing processes or ways of thinking, to confirm or refute current ways of seeing, to generate new lines of enquiry or make links where none had been previously considered. The vast majority of scholarly research is 'incremental'; most scholarly papers are focused, sometimes very narrowly, on very specific aspects of a research subject. Text mining offers the possibility of being able to overview, identify and extract the information that enables a cumulative picture of the many thousands of incremental increases in knowledge that each scholarly paper represents, a cumulative impact that can lead to innovation.
The nature of text mining makes it impossible to put 'hard figures' on its potential future value to the economy through the innovations it may engender; the BIS strategy view on the development of new technologies in the innovation process could easily describe the role of text mining.
'The future of new technologies is inherently uncertain in part because success often depends on extended process of multiple innovations, which shapes and expands new application areas and generates returns. Increases in productivity growth tend to be produced over time by the cumulative effect of a series of improvements within a new technological system, rather than by a single innovation. It is very challenging to foresee the trajectory of future improvements and quantify in advance the economic benefits those improvements will generate' [177]
5.2.2 The potential for new cutting edge services and business models
Research using text mining requires an extensive range of supporting infrastructure and services. This includes domain-specific tools, training and the construction and curation of collections of documents that are in compatible formats for mining. As there is a strong predominance of English language journals in the scholarly publishing world this also gives an advantage to the UK for capitalising on demand for text mining. The time could come when UK-developed text mining tools and services become essential purchases alongside any journal access licences and create opportunities for new service development, which the UK's leading publishing industry is well placed to take.
Smit and Van der Graf found that the 'new service' businesses they consulted were among the most optimistic of their participants about the possibilities for developing the new services that a body of academic text miners would need [178].
Certainly the rapid growth and development of firms such as Mendeley, as shown in the case study 4.5 is evidence of potential for new business innovations surrounding the research market. As the case study shows, Mendeley chose to locate in the UK because of strong research universities, the English language base, good access to venture capital and a thriving publishing industry.
Overall, there are strong indicators that text mining could make an important contribution to stimulating and supporting innovation.
5.3 Market failure and fairness
Current copyright law-driven restrictions on text mining, particularly the text mining of scholarly journals, appear to be inhibiting its wider usage or take-up in UKFHE. Without wider usage, the potential for text mining to generate gains for the economy and society cannot be exploited and the UK economy will be less able to take advantage of its strong public research base. This carries dangers of 'being left behind' as other competitor countries (such as Japan) adopt a more liberal approach that encourages text mining usage.
This observation raises some fundamental questions about the nature and structure of the market for text mining of scholarly journals and why such a situation exists. The current situation may be a result of market failure, which would be detrimental to the economy and society overall. Consideration of the value chain of the scholarly publishing communication system, which shows a substantial public investment in the underlying research base, also raises the question of 'equity' or fairness in current market operations: are copyright barriers to text mining in UKFHE preventing society from deriving a fair share of the return on society's own investment in research? [179]
The issue of market failure is a complex one, involving consideration of the fundamental theory of welfare economics as well as economics [180] of property rights [181]. In the following discussion, the concept of market failure is first discussed. Its four key indicators are then explained and examined in relation to the key issues (highlighted in the consultations, case studies and desk research). This is important because if there are indicators of market failure in relation to text mining use this would support the case for the Hargreaves recommended copyright exception. Conversely, if there is little or no significant market failure indicated, there would be no reason to intervene – solutions are likely to emerge over time.
The exploration adheres closely to the definition of 'market failure' and reasons for government intervention in the market as provided in the UK Treasury Green Book [182,183].
These reasons are:
To improve the achievement of economic objectives by addressing 'market failure'
To address issues of 'equity' or fairness
5.3.1 Market failure
The Green Book explains that 'market failure' occurs when the usual market mechanisms and transactions do not enable the achievement of 'economic efficiency'. Economic efficiency is the 'ideal' state when all relevant resources are being allocated and used to their maximum productivity: the point is reached where no one can become better off without someone else becoming worse off [184].
However, the real world is not perfect; for a variety of reasons markets do not always achieve this ideal state and are said to 'fail.' This can frequently be the case if there is a mismatch or imbalance between the returns to society as a whole from an activity and the returns to the private individual or organisations involved. Such an imbalance can affect motivations and behaviours in ways that negatively affect the market outcomes and mean that the outcomes are not the best that can be achieved for the economy and society.
The 'Green Book' highlights that this may happen for a number of possible reasons:
- Due to the 'public good' characteristics of the goods or service under consideration
- Where there may be significant 'externalities' (positive or negative) involved
- Where there is imperfect information or information asymmetry between buyers and sellers
- As a result of market power or structure (eg a lack of competition, monopoly power or high entry costs deterring entrants)
For each of these criteria in turn, text mining is examined against them to explore if there are possible indicators of market failure in relation to text mining or text mining usage.
Public good characteristics
The first indicator of potential for market failure is if the goods or services have 'public good' or 'quasi-public good' characteristics. This does not mean that they are anything to do with the public sector. 'Public goods' are typically ones that are difficult to 'trade' in a market place. They are defined as being 'non-rivalrous' and 'non-excludable'.
Put simply, this means that once such a good exists, it is hard to prevent it from being used (eg clean air) and also one person's usage does not necessarily prevent others' usage. (A classic example is looking at a lighthouse – but looking at a digital photograph is another modern example.) This presents problems for trading as it means there is a 'free rider' problem – if, once a good is produced, there is no way to prevent anyone benefitting from it, individual consumers will not pay for it although they are enjoying the benefits. In this case there is less incentive for private producers to provide the good so there is likely to be an undersupply.
Does text mining have public good characteristics?
- Text mining is clearly non-rivalrous. Researcher A undertaking a text mining process does not reduce the potential for researcher B to undertake the same text mining process or indeed it makes no difference if 1,000 researchers do so. At the margins there might be some issue relating to storage capacity if large numbers of researchers need data storage facilities, but in principle, in a digital world, the mining of text is non-rivalrous.
- Is text mining excludable? Under current legislation, text mining can be excludable (and indeed many of the barriers identified by participants relate precisely to its excludability). One must possess text mining tools, but importantly one can be excluded and prevented from text mining the corpora of documents by having electronic access terminated by the copyright holder or content provider.
This presents an interesting conundrum. Text mining currently displays some public good characteristics (non-rivalrous) but text mining does not display across the board public good characteristics because it has evolved in a world where pre-existing copyright legislation can make it excludable. This suggests that it may in fact have fundamental public good characteristics – without the existence of copyright restrictions it would be non-excludable. Indeed, where there are no such restrictions (e.g. the mining of Open Access or non-copyright material), text mining is non-excludable i.e. it would be difficult to prevent people from undertaking it if they wished.
Therefore text mining appears to possess at least some degree of public good characteristics, which suggests the potential for market failure – ie less text mining would take place than would be in the economic interests of society as a whole.
Externalities
Externalities can be described as 'third party effects', additional (sometimes accidental) impacts on other people who are not directly involved in the original set of actions or transactions. Externalities can be positive or negative.
- A 'text book' example of a positive externality is that of the bee keeper, whose aim is to produce honey, but whose bees also pollinate the surrounding orchards, thereby assisting the fruit-growers to produce crops.
- A 'text book' example of a negative externality is that of pollution; the discharges from a factory may pollute a river which kills the fish and impacts on the livelihoods of fishermen downstream. Excessive car fumes may pollute the air and be detrimental to the health of people in a heavy traffic area.
Externalities can be a source of market failure because they cause a divergence between social costs and benefits and private costs and benefits. Positive externalities can be an indicator of market failure precisely because these benefits are accruing to third parties who are not involved in the initial activities. It may be socially desirable to have more of them but there is no incentive for the actively involved private parties (for whom the externalities may be an irrelevance) to produce more of these benefits, so there may be less produced than would be ideal.
Conversely negative externalities can be an indicator of market failure if the 'third party effects' are excessively detrimental – but there is no immediate incentive for the directly involved parties to change their behaviour so more of these effects are produced than is ideal.
The impact of such externalities may be sufficiently significant to justify intervention. The 'polluter pays' principle, which is used to justify a range of 'green' taxes, is based on the notion that pollution is a negative 'externality' and intervention is justified to mitigate its effects.
Are there externalities to text mining usage?
Text mining is simply an electronic process to support more efficient and more extensive research. In itself text mining may generate externalities if the process has other, broader, consequences unrelated to its purpose, for instance negative environmental consequences from the use of servers and equipment or positive environmental consequences from reducing 'paper mountains'. But these are not likely to be significant; the environmental impact of one may balance out the environmental impact of the other.
The real question of whether text mining in UKFHE would generate externalities (positive or negative) is related to the extent that non-commercial academic research is believed to generate wider impact on society. If text mining enables a more extensive body of research or generates new knowledge or insights that are of benefit to society and the economy more generally, rather than directly benefiting the person or organisation undertaking the text mining, then it could be said to generate positive externalities. Much of the evidence relating to the role of the university research base in stimulating innovation is linked to the idea that research has a wider impact beyond those immediately involved This includes a substantial body of literature that considers the existence of 'knowledge spillovers', including 'agglomeration effects', such as clustering [185], as positive externalities to university research [186, 187]. Therefore text mining usage in research is likely to have a range of positive externalities which means market failure may arise.
Imperfect information
A 'functional' market is regarded as being dependent on those concerned in a transaction having sufficient information about a product or service to make well-informed decisions about it. If one side knows considerably more, there is an imbalance or asymmetry which can lead to market failure: some products may be under-produced and under consumed, others may be over-produced and over consumed.
There appears to be a high degree of uncertainty and a lack of knowledge about the implications, value, ultimate outcomes or impact of text mining in research
There appears to be a high degree of uncertainty and a lack of knowledge about the implications, value, ultimate outcomes or impact of text mining in research, both on the part of potential text mining users and on the part of copyright holders. This is further complicated by high transactions costs, which place a heavy burden on potential users.
The Smit and Van der Graf report on journal text mining [188] highlighted that lack of knowledge about text mining was a problem for publishers and content providers:
'From the interviews it became clear that knowledge about content mining is often fragmented and thinly spread throughout the larger publishing organisations and that policy making on content mining in most publishing organisations is still very much in development'.
The evidence from this study has also highlighted that text mining in UKFHE is relatively restricted to a number of specialist groups, with low levels of knowledge about text mining outside those groups. There is limited 'hard' information on the benefits and outcomes of text mining because there needs to be more widespread take-up and use to observe such benefits.
Text mining has similar characteristics to those of basic or 'blue skies' research (indeed in many cases it will in fact be undertaken as part of basic research); the text mining outcome cannot be predicted in advance and there is therefore uncertainty about the downstream value or impact of any specific instance of it. Indeed, in seeking permission to undertake text mining of a body of text there is not a great deal that can be said beyond indicating the broad purpose of the text mining request. This can cause problems in obtaining permission for its use [189], which adds to the already heavy burden of transactions costs.
Evidence from our consultation and from that presented to Hargreaves [190] indicated high transaction costs arising from the extremely time consuming and resource intensive process required to navigate the different journal licensing arrangements (see the Wellcome case in 4.2). But the risks of not seeking permission are substantial (as all access to the licensed content could be 'pulled' if unauthorised mining is detected.)
The 2011 Smit and Van der Graaf survey of scholarly publishers confirmed the existence of high transactions costs and highlighted a very diverse picture of polices and permissions for text mining. A proportion, mainly Open Access publishers, said they have no restrictions on text mining; a minority had a clear publicly available permissions policy; and the majority indicated that permission for text mining was considered on a case by case basis. Of the latter just over one-third (34.6%) said they generally (but not always) grant permission, 52.9% said they sometimes do and 12.5% said they never do. Around 2/3 respondents said they would tend to grant permission for research purposes but the remaining third said they would only do so in some cases.
The complexities of seeking access permission for text mining were stressed in a number of submissions to Hargreaves e.g. [191], and this may be reflected in the Smit et al finding that in practice publishers received relatively few requests for permission to text mine from academic researchers (around half of the respondents indicated they had received requests but typically fewer than five per year).
Smit and Van der Graf [192] comment:
"in the discussions around text mining, the impression is frequently left that there is a huge demand from individual re-searchers to mine more and deeper. But publishers see very few of those requests really reach their desk. This poses the question whether it is now too complicated for individual researchers to know how to deal with publishers about this (so many different publishers to ask and what is the procedure) or where the demand is in fact lower than the content mining community wishes to believe."
The information deficit and the high transaction costs associated with text mining may well result in market failure; high transaction costs and uncertainty reduce demand potential and without demand potential there is less likelihood of any re-turn on investment in tools and services to support text mining, which inhibits innovation in services to support text mining. This is also likely to hinder the development of satisfactory 'licensed solutions'. BIS has recognised that the lack of clear information concerning the demand and particular needs of a market is an obstacle to licensed solutions.
'Where licensing of copyright content is targeted at a well-defined group need, with good guidelines and prices that can be arbitrated, licensing systems have evolved to allow the public to use copyright material – as with schools, universities etc. Where such a distinct group does not exist, licensing has been slow to emerge and copyright content remains locked away, preventing possible innovation and exploitation of copyright assets [193].
The information deficit and high transactions costs surrounding text mining in research are strongly suggestive of a situation of market failure.
Market power
The final criterion cited by the 'Green Book', which has potential to create market failure, is 'market power'. Put simply, this could describe a situation where there is over dominance in a market, which inhibits the functioning of a competitive market – where, for example, there is a dominant or single (monopolistic) seller or a dominant or single (monopsonistic) buyer. As the 'Green Book' itself states it can also include a scenario where there are high entry or start-up costs that reduce potential competition.
Text mining in UKFHE research is not a 'stand-alone' activity but is embedded in the complex landscape of scholarly journal licensing and permissions. It is about the additional use and exploration of electronic content for which the user has typically already purchased a licence. Therefore restrictions on its use are bound up with the more general operations of the scholarly journal market and the application of copyright and contract law. The existence of copyright (which confers a 'monopoly right' on the holder [194, 195]) clearly creates a special position in this market and makes the question a complex one.
On the face of it, the scholarly journal market appears to be highly competitive, which suggests a functioning market. According to the STM Report [196] there are estimated to be around 2,000 journal publishers across the globe, publishing over 25,000 peer-reviewed journals with around 1.5 million articles per year. The main English language trade and professional associations for journal publishers account for nearly 50% of total journal output (11,550 journals from 657 publishers).
Likewise, UKFHE institutions are not 'monopsonistic' buyers. There are over 165 higher education institutions in the UK, and more than 400 further education institutions. Journal publishers serve a global market selling to higher and further education institutions, libraries and research institutions around the world as well as to private companies and firms. In addition to this, there is a growing trend for articles and research outputs to be made freely available through Open Access routes, such as institutional repositories.
However, there are some caveats, which may at times create a situation of market power; it should be noted that there are a number of subject 'niches' and specific high profile and high 'research rated' or 'high impact' journals that cannot simply be substituted by the purchaser with another one that is 'cheaper' or 'better value' or 'less restrictive in licensing terms'. One should also note the existence of a number of large journal publishers who have extensive portfolios covering a consider-able portion of the market. The top ten publishers are responsible for about 35% of journals and four publishers50 have over 1,000 titles each. This gives those large publishers a strong market influence.
Overall, however, there does not appear to be evidence for market failure arising from a predominance of market power.
Indicators for existence of market failure | Text mining usage in UKFHE research | Comments |
---|---|---|
Public good characteristics | Yes | Non-rivalrous |
Externalities | Yes | Wider social and economic impact arising from research: knowledge spillovers |
Imperfect information | Yes | on both sides |
Market power | No | Some caveats with existence of a small number of majot players |
Figure 5 presents the outcome of the assessment of the evidence for indicators of market failure. There appears to be strong evidence for market failure against three of the four indicators for market failure in the 'Green Book'. Strong evidence for market failure can justify government intervention and would tend to support the case for the Hargreaves recommended copyright exception on the grounds of improving economic efficiency.
5.4 Equity: who pays and who gains?
A number of those interviewed for this study drew attention to the significant investment embodied in the corpora of data being mined ie the actual research base itself. While the text mining process had particular costs associated with it (including equipment, software, training, licensing and curation costs), these paled into insignificance beside the underlying investment that had been made in the original research itself. The additional costs associated with text mining would be a small price to pay if they enabled the leverage of maximum value from the existing research base.
The 2008 CEPA study [197] of the scholarly communications system highlighted that the majority of the investment in the scholarly communications system was located in the underlying research production stage, with user consumption of articles the next most intensive stage (see section 5.1, Figures 3 and 4). Publishing and distribution, although critical to the overall process, accounted for only a 'small part' of the overall costs.
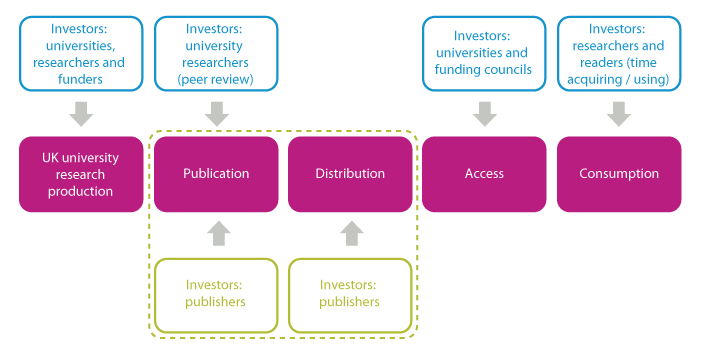
Figure 6: Value chain of the scholarly communications process
Text description for figure 6
Value chain of the scholarly communications process
Investors: universities: researchers and funders - UK university research production
Investors: university researchers (peer review) - publication and distribution (with investors: publishers)
Investors: universities and funding councils - access
Investors: researchers and readers (time acquiring/using) - consumption
Value chain of the scholarly communications process
Investors: universities: researchers and funders - UK university research production
Investors: university researchers (peer review) - publication and distribution (with investors: publishers)
Investors: universities and funding councils - access
Investors: researchers and readers (time acquiring/using) - consumption
The pattern of investment and cost flows within the scholarly communications value chain is important because, as Figure 6 shows, in the UK the majority of research (the production) undertaken in UKFHE is publicly funded; public investment in research is channelled to and through the higher education sector. There is also a significant proportion of charitable or philanthropic funding. The public purse is also involved in other parts of the value chain, in enabling access to the content both through higher education institutions and separately [198].
Is the level of public investment significant?
HESA data show that in 2009/2010 over £6.3bn was invested in UK higher education research infrastructure and research projects. As Figure 7 illustrates, 68% of this was public money, through the funding councils, the research councils and other government bodies such as the National Health Service and central, devolved and local government. Another 15% came from UK charities, with 4% from the UK private sector and the remainder from non-UK sources (including EU government bodies and charities).
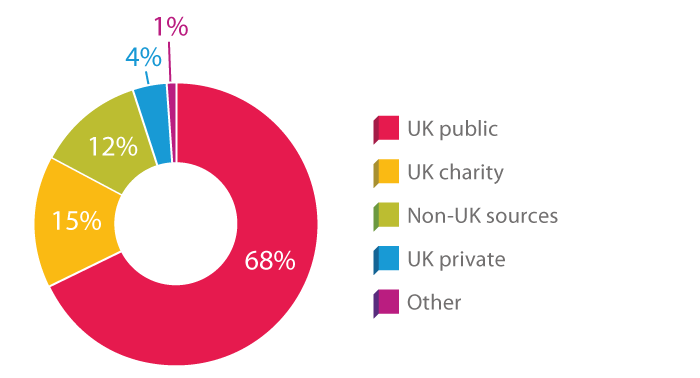
Figure 7: Research funding in UK higher education institutions 2009/2010. Includes both recurrent research grant from the funding bodies (£1.9bn) and income for research grants
Text description for figure 7
Research funding in UK higher education institutions 2009/2010
- 68% UK public
- 15% UK charity
- 12% non-UK sources
- 4% UK private
- 1% other
Research funding in UK higher education institutions 2009/2010
- 68% UK public
- 15% UK charity
- 12% non-UK sources
- 4% UK private
- 1% other
There is increasing demand from public and charitable funders that maximum value is leveraged from their substantial investment and this includes making outputs accessible and usable. The new government innovation strategy [199] is proposing increased availability of publicly-funded datasets and increased access to publicly-funded research. As part of this policy drive the research councils have undertaken to enforce their current policy of making all outputs from the work they fund openly accessible. They are creating a new 'Gateway to Research' where research outputs are made available in a common and reusable format [200].
Privately erected barriers by copyright holders that restrict text mining of the research base could be increasingly regarded as inequitable or unreasonable since the copyright holders have borne only a small proportion of the costs involved in the overall process; furthermore, they do not have rights or ownership of the inherent facts or ideas within the research base.
Text mining offers the potential for fuller use of the existing publicly-funded research base. Privately erected barriers by copyright holders that restrict text mining of the research base could be increasingly regarded as inequitable or unreasonable since the copyright holders have borne only a small proportion of the costs involved in the overall process; furthermore, they do not have rights or ownership of the inherent facts or ideas within the research base.
An important point that emerges here is that the value chain for scholarly journals and the balance of investment, risk and reward is very different from the value chain for other copyright products. Economic arguments in support of copyright that are valid for other creative products do not apply in the same way to scholarly journals.
The PricewaterhouseCoopers report on the economics of copyright [201] gave an exposition of the value chain for creative content as it pertained to the example of a book. This demonstrated how copyright supported the generation of incentives and rewards for creators and developers, with rewards for the author coming through initial sale or licensing of their creative work to a publisher and then further reward through a share of secondary licensing revenues (through licensed copying of the work). The publisher invests in the author's work, paying the author for the rights and covering the risks and costs of taking it to market. There is a balance of investment and risks between the content producer and the publisher and a negotiated balance of risks and rewards.
However, the value chain for scholarly journals and the text mining of scholarly journals is significantly different, involving many more players and investors and the share of the costs and risks burden is very different. As the CEPA analysis [202] has shown, the publisher investment in the scholarly communications process is an important but relatively small overall part of the investment required. The majority of the investment and risk burden is borne by the public purse, philanthropic funders and researchers and users themselves. Hence, the broader interests of equity may support the case for an exception to enable text mining so that society can maximise the potential returns from an asset in which society has made the lion's share of investment and taken the vast majority of the risk.
5.5 Reflections on the economic assessment of text mining in UKHFE
- We have found evidence for a clear potential for text mining usage in UKFHE to generate significant productivity gains, with benefit both to the business of the sector itself and to the wider economy
- Widespread take up of text mining by higher education researchers could be an opportunity for the UK, encouraging innovation and growth through leveraging additional value from the public research base
- The UK has a number of strengths including good framework conditions for innovation and the natural advantage of its native language for it potentially to be an early mover in text mining development. The scholarly publishing market is a global market with global potential for demand for text mining tools and services. This offers opportunities for new service companies as well as current content providers
- However, these opportunities for productivity improvements, knowledge discovery and innovation are being hindered by a range of economic-related barriers including legal restrictions, high transactions costs and information deficit, which are strongly indicative of market failure
- Text mining offers new opportunities for knowledge discovery and generation. The technological developments that would make this possible are recent and were not envisaged in previous consideration of the impact of copyright. However, because the technical process of text mining involves the production and storage of copies of material that may be subject to copyright, there is a new conundrum: the market intervention of copyright, originally intended to protect creative producers, is itself becoming a barrier to new creative production and may be inhibiting new knowledge discovery and innovation
- When the use of text mining is examined in the context of the key recognised indicators for market failure, the available evidence suggest that there may be a degree of market failure involved, possibly a very significant degree
- When equity issues are taken into consideration there are further signs that the interests of society as a whole may not be well served by the current limitations on text mining
6. Summary of findings
The potential for text mining and text analytic technologies and practices in UKFHE
Where it is being used, text mining and analytics are being successfully employed in research to generate new knowledge and to support the research process
- Text mining offers a way of helping researchers to make sense of and leverage value from the vast sea of electronic resources, which is continually expanding. These research resources include both raw information sources such as the web and extant scholarly communications
- There is significant potential for using text mining to facilitate and advance research across all disciplines in UKFHE
- Use is most advanced within the biomedical sciences and related fields. Much of this work has involved development of and experimentation with text mining tools to explore their potential applications within the domain. However, text mining in these fields is beginning to be embedded in some workflows, which will aid uptake
- Use within other fields in UKFHE is less widespread, although pilot initiatives are beginning to explore its possibilities
- Where it is being used, text mining and analytics are being successfully employed in research to generate new knowledge and to support the research process
- Text mining and analytics have the potential to increase the research base available to business and society and to enable business and others to use the research base more effectively
- However, access restrictions to copyrighted documents, transaction costs, entry costs, lack of open infrastructure and lack of critical mass are all barriers to uptake
- Participants and evidence from the case studies suggest that barriers to uptake and restrictions in use of text mining and analytics that are limiting uptake have wider implications in terms of hindering innovation
The costs, benefits (in particular the economic value) and risks of exploiting text mining, both now and in the foreseeable future
- There is a range of costs associated with text mining. These relate to access rights to text-minable materials, transactions costs (participation in text mining), entry (setting up text mining), staff and underlying infrastructure. Currently, the most significant costs are transaction costs and entry costs
- Given the sophisticated technical nature of text mining, entry costs will remain high, although the entry costs associated with some simpler text mining tools can be expected to reduce
- High transaction costs currently are attributable to uncertainty surrounding permission for text mining of the collections that researchers wish to study and the need to negotiate a maze of many and diverse licensing agreements covering the collections researchers wish to study. These costs are currently borne by researchers and institutions, and are a strong hindrance to text mining uptake. These could be reduced if uncertainty is reduced, more common and straightforward procedures are adopted across the board by license holders, and appropriate solutions for orphaned works are adopted. However, the transaction costs will still be significant if individual rights holders each adopt different licensing solutions and barriers inhibiting uptake will remain
If use of text mining were to increase significantly, a corresponding infrastructure would need to be developed to support its use. - These costs could be significant; however, if large pooled infrastructures were developed, efficiencies of scale would reduce the overall costs
- Research benefits include: efficiency; unlocking hidden information and developing new knowledge; exploring new horizons; improved research and evidence base; and improving the research process and quality
- Broader economic and societal benefits include: cost savings and productivity gains; the potential for new radical and incremental innovation with wider economic benefit and including innovative service development; new business models and potential for research discoveries and applications of widespread significance such as medical advances
The main barriers to the exploitation of this potential, and how might they be overcome
- Participants and case studies in general felt that there were significant barriers to uptake of text mining in UKFHE
- The barriers and risks include: uncertainty regarding the legality of text mining; entry costs; 'noise' in results; document formats; information silos and corpora specific solutions; lack of transparency; lack of support, infrastructure and technical knowledge; lack of critical mass; and mass exclusions from collections due to misuse of others
- Participants and case studies suggested a number of developments that could help overcome many of the barriers, including:
- A text mining exception to copyright
- Standardisation of metadata and collection formats
- A move towards Open Access to scholarly publications
- Better support for novice users
- More information about use and potential benefits
- A more critical mass of users within their field
Cost savings and productivity gains related to text mining
- The evidence gathered shows that there is clear potential for text mining usage in UKFHE to generate significant productivity gains, with benefit both to the business of the sector itself and to the wider economy
- Widespread take up of text mining by higher education researchers could be an opportunity for the UK, encouraging innovation and growth through leveraging additional value from the public research base
Wider impact on the economy and innovation system
- The UK has a number of strengths, including good framework conditions for innovation and the natural advantage of its native language for it potentially to be an early mover in text mining development. The scholarly publishing market is a global market with global potential for demand for text mining tools and services. This offers opportunities for new service companies as well as current content providers
- However, these opportunities for productivity improvements, knowledge discovery and innovation are being hindered by a range of economic-related barriers, including legal restrictions, high transactions costs and information deficit
Market efficiency and equity
- Text mining offers new opportunities for knowledge discovery and generation. The technological developments that would make new knowledge creation possible are of relatively recent development, and hence were not envisaged in previous consideration of the impact of copyright. However, because the technical process of text mining involves the production and storage of copies of material that may be subject to copyright, there is a new conundrum: the market intervention of copyright – originally intended to protect creative producers – is becoming in itself a barrier to new creative production and may be inhibiting new knowledge discovery and innovation
- When text mining usage is examined in the context of the key recognised indicators for market failure, the available evidence suggest that there is a degree of market failure involved, possibly a very significant degree
- When equity issues are taken into consideration there are further signs that the interests of society as a whole may not be well served by the current limitations on text mining
6.1 Limitations and issues
As highlighted in the introduction (section 1.4), the short time scales, small scale of the project and the limited use of text mining in UKFHE restricted the evidence, particularly the quantitative data, that could be collected. Participation in the study was further limited by two more sensitive reasons. First, some of the text mining that is currently undertaken might not necessarily abide by strict copyright licensing agreements and, second, the qualitative data relating to use can be considered commercial sensitive. This data limitation impacted the study in two ways. First, it meant that case studies needed to be stylised, combining existing and potential use cases, drawing on otherwise analogous uses in, for example, the commercial sector. Second, while best effort has been made to draw appropriate generalisations based on the case studies and quantitative data relating to research practice, these generalisations are indicative rather than statistically relevant. However, they provide a reasonable indication of the scale and magnitude of the economic benefits that could be derived.
A further significant limitation is that the exploitation of text mining in UKFHE is inexorably linked with the operations of the scholarly publication system. It is therefore difficult to examine text mining in isolation and some of the costs and barriers identified are not specific to text mining alone.
7. Conclusions and recommendations
7.1 Economic and regulatory related
There is evidence to suggest a degree of market failure in text mining. There are also fundamental questions about the 'fairness' of the current situation that limits text mining usage in UKFHE and thereby limits the returns to society. This would tend to support the Hargreaves recommendation for an exception to text mining for non-commercial use.
Recommendation 1:
Policymakers should consider the evidence for market failure and issues of equity relating to text mining and current copyright law that is highlighted in this report.
New business models for supporting text mining within the scholarly publishing community are being explored; however, evidence suggests that in some cases lack of understanding of the potential is hampering innovation.
Recommendation 2:
The UKFHE sector collaborates with content publishers and service providers to explore potential new business models and innovative text mining services that meet the sector's requirement.
The consultations and case studies suggest that text mining is currently extremely limited within UKFHE, in part at least due to the current licensing arrangements. A text mining exception, if it were to be implemented, would remove a key barrier thus better enabling service solutions supporting text mining to emerge from the market.
Recommendation 3:
An exception to support text mining and analytics for non-commercial research, such as the one suggested by Hargreaves, should be implemented.
It is not possible to consider text mining of scholarly journals in isolation from the overall operations of the scholarly communications system. In exploring issues of market failure and equity in relation to text mining, it appeared that similar fundamental issues (in particular the matter of equity in terms of 'who pays and who gains') may be relevant to the overall debate surrounding Open Access to scholarly outputs. This point is very specifically about the economics of copyright applied to scholarly journal publishing, which has a range of differences from other parts of the publishing industry.
7.2 Infrastructure and support related
Realisation of the full potential of text mining within UKFHE is inexorably linked to the scholarly publication system. Issues relating to interoperability, information silos and access restrictions are limiting the uptake, degree of automation and potential application areas of text mining.
There is a significant lack of awareness regarding the potential for text mining in research apart from in specialised fields. This is hindering uptake.
Recommendation 4:
Work needs to be undertaken to raise awareness of the potential capabilities and value of text mining to UKFHE. A useful starting point might be to conduct surveys of UKFHE and key agencies such as the research councils, Higher Education Academy and a range of scholarly societies to ascertain current levels of awareness.
Researchers require better advice on the benefits of text mining and the practicalities of incorporating it into their research practice. This includes advice on tools and techniques, costs and obtaining permission to text mine where required.
Recommendation 5:
Advice and guidance should be developed to help researchers get started with text mining. This should include: when permission is needed; what to request; how best to explain intended work and how to describe the benefits to research and copyright owners. Relevant advice and training should be available for postgraduate students.
It is questionable whether the current infrastructures and support systems that underpin text mining will scale should uptake increase significantly within UKFHE. Exactly how text mining usage evolves will depend on whether Hargreaves' recommended exception is implemented. However, it is important that UKFHE achieves a cost-effective solution that meets researchers' needs.
Recommendation 6:
Once the decision has been made regarding Hargreaves recommended exception, Jisc should work with scholarly publishers, technology service providers and other key stakeholders to explore the technical requirements for optimal provision of text mining infrastructure services. This should include a focus on interoperability and metadata standards.
Appendices and acknowledgements
Acknowledgements
The authors would like to thank:
Attendees at the peer review workshop 10 February 2012 John Watts, AHRC; Naomi Korn, Copyright Consultant; Stuart Dempster and Torsten Reimer, Jisc; Victor Henning, Mendeley, Vic Lyte, MIMAS; John McNaught, NaCTeM; Stephen Pinfield, University of Nottingham; and David Carr, Wellcome Trust
The following individuals for their valuable input: Project mentors Professor Iain McNicoll and Dr George Weir, University of Strathclyde; Vic Lyte, MIMAS; Dr Victor Henning, Mendeley; Dr Claire Grover and Dr Bea Alex, University of Edinburgh; Professor Douglas Kell, University of Manchester; and The Wellcome Trust. The following organisations for participating in consultations:
- Abertay University
- British Library
- Coadec
- Digging into Data Challenge
- Digging into Data Competition
- Jisc
- Kings College London
- Mendeley
- MIMAS
- National Centre for Text Mining (NaCTeM)
- Publishers Association
- Startup Intelligence
- University of Edinburgh
- University of Manchester
- University of Strathclyde
- UK PubMed
The authors would like to thank:
Attendees at the peer review workshop 10 February 2012 John Watts, AHRC; Naomi Korn, Copyright Consultant; Stuart Dempster and Torsten Reimer, Jisc; Victor Henning, Mendeley, Vic Lyte, MIMAS; John McNaught, NaCTeM; Stephen Pinfield, University of Nottingham; and David Carr, Wellcome Trust
The following individuals for their valuable input: Project mentors Professor Iain McNicoll and Dr George Weir, University of Strathclyde; Vic Lyte, MIMAS; Dr Victor Henning, Mendeley; Dr Claire Grover and Dr Bea Alex, University of Edinburgh; Professor Douglas Kell, University of Manchester; and The Wellcome Trust. The following organisations for participating in consultations:
- Abertay University
- British Library
- Coadec
- Digging into Data Challenge
- Digging into Data Competition
- Jisc
- Kings College London
- Mendeley
- MIMAS
- National Centre for Text Mining (NaCTeM)
- Publishers Association
- Startup Intelligence
- University of Edinburgh
- University of Manchester
- University of Strathclyde
- UK PubMed
Footnotes
- M. Ware and M. Mabe, "The stm report: An overview of scientific and scholarly journal publishing," 2009.
- I. Hargreaves, "Digital Opportunity: A Review of Intellectual Property and Growth," 2011.
- HM Treasury, The Green Book: Appraisal and Evaluation in Central Government, no. July. 2011, pp. 57-58.
- J. Manyika et al., "Big data?: The next frontier for innovation , competition , and productivity," 2011.
- Facebook users share over 30 million pieces of content per month [3] and twitter has 350 million tweets daily
- J. Manyika et al., "Big data : The next frontier for innovation , competition , and productivity," 2011.
- The MGI study focuses specifically on what it terms 'Big Data' datasets whose size is beyond the ability of typical database software tools to capture, store, manage, and analyse
- MoneyWeek, "How Tesco became Britain's top supermarket," 2007. [Online. Accessed: 15-Feb-2012.]
- S. Grimes, "Text-Analytics Demand Approaches $1 Billion," InformationWeek, 2011. [Online. Accessed: 30-Nov-2011.]
- ScraperWiki, "ScraperWiki." [Online. Available: https://scraperwiki.com/ [Accessed: 30-Nov-2011.
- CommonCrawl, "CommonCrawl." [Online. Available: http://www.commoncrawl.org/ [Accessed: 30-Nov-2011.
- E. Smit and M. Van der Graaf, "Journal article mining," 2011. Available: http://web.archive.org/web/20140102230201/http://publishingresearch.net/ [Accessed 13-Mar-2012
- N. R. Smalheiser and D. R. Swanson, "Indomethacin and Alzheimer's disease," Neurology, no. 46, p. 583, 1996.
- Gartner Inc., "Gartner Identifies the Top 10 Strategic Technologies for 2012," 2011. [Online.]
- I. Hargreaves, "Digital Opportunity: A Review of Intellectual Property and Growth," 2011.
- Compare www.cs.cmu.edu/~dunja/CFPWshKDD2000.html with http://web.archive.org/web/20120303042253/http://www.ir.iit.edu/cikm2004...
- UKPMC, "UK PubMed Central." [Online. Available: http://ukpmc.ac.uk/About.
- Institute of Education, "The UK Educational Evidence Portal," 2009. [Online. Available: http://www.eep.ac.uk/dnn2/tabid/36/Default.aspx [Accessed: 12-Feb-2012.
- Office of Digital Humanities, "The Digging into Data Challenge," 2012. [Online. Available: http://www.diggingintodata.org/ [Accessed: 21-Jan-2012.
- S. Ananiadou, J. Chruszcz, J. Keane, J. McNaught, and P. Watry, "The National Centre for Text Mining: Aims and Objectives," Ariadne, no. 42, 2005.
- K. Allman et al., "Measuring Wider Framework Conditions for successful innovation: A system's review of UK and international innovation data," 2011.
- U. Kelly, D. McLellan, and I. McNicoll, "The Impact of Universities on the UK Economy," 2009.
- In.Dig.O, "Analysis of the Value and Benefits of Text Mining and Text Analytics to UK HE and FE." [Online. Accessed: 21-Jan-2012.]
- I. Hargreaves, "Digital Opportunity: A Review of Intellectual Property and Growth," 2011.
- HM Treasury, The Green Book: Appraisal and Evaluation in Central Government, no. July. 2011, pp. 57-58.
- P. A. Champ, K. J. Boyle, and T. C. (Eds. . Brown, A Primer on Nonmarket Valuation. Kluwer Academic Publishers, 2003.
- HM Treasury, The Green Book: Appraisal and Evaluation in Central Government, no. July. 2011, pp. 57-58.
- A fifth stage – exploring exploitation (barriers, solutions and resources) – had been planned; however, this was rolled into the consultation and case study phases. Further details of the study approach can be found on the project website
- While anonymised case studies were considered to encourage participation, it was clear that it would be difficult then to meet the IPO's evidence criteria
- HM Treasury, The Green Book: Appraisal and Evaluation in Central Government, no. July. 2011, pp. 57-58.
- HM Treasury, The Magenta Book: Guidance for evaluation. 2011.
- HM Treasury, "The Orange Book: Management of Risk- Principles and Concepts," 2004.
- Intellectual Property Office, "Good Evidence for Policy," 2012. [Online. Available: http://webarchive.nationalarchives.gov.uk/20140603093549/http://www.ipo.... [Accessed: 16-Jan-2012.
- Office of Digital Humanities, "The Digging into Data Challenge," 2012. [Online. Available: http://www.diggingintodata.org/ [Accessed: 21-Jan-2012.
- HathiTrust Research Center, "HathiTrust Research Center." [Online. Available: http://www.hathitrust.org/htrc [Accessed: 15-Feb-2012.
- Eigenfactor.org, "Eigenfactor.org." [Online. Available: http://www.eigenfactor.org/about.php [Accessed: 15-Feb-2012.
- T. J. Larsson, M. Jansson, and B. Brooks, "TEXT-MINING OF INSURANCE-BASED INFORMATION: DECISION SUPPORT FOR LOCAL SAFETY MANAGEMENT," Safety Science Monitor, no. 1, pp. 1-9, 2009.
- University of Tokyo, "Tsujii Laboratory." [Online. Available: http://web.archive.org/web/20140722105856/http://www.nactem.ac.uk/tsujii/ [Accessed: 15-Feb-2012.
- MacQuarie University, "Centre for Language Technology." [Online. Available: http://clt.mq.edu.au/ [Accessed: 15-Feb-2012.
- Ben-Gurion University, "Text Mining Day 2009." [Online. Available: http://www.cs.bgu.ac.il/~frankel/TextMiningMay09/abstracts_bios.html [Accessed: 15-Feb-2012.
- Fraunhofer AIAS, "Text Mining and Information Retrieval." [Online. Available: http://web.archive.org/web/20130117005356/http://www.iais.fraunhofer.de/... [Accessed: 15-Feb-2012.
- Chinese Academy of Sciences, "Chemical Informatics Improved Text Mining." [Online. Available: http://web.archive.org/web/20121011070350/http://english.cas.cn/Ne/ICN/2... [Accessed: 03-Feb-2015.
- M. Ware and M. Mabe, "The STM Report: An overview of scientific and scholarly journal publishing," 2009.
- A broad key-word search could potentially bring more journals to the attention of the researcher than (s)he would have looked at manually.
- L. Hawizy, D. M. Jessop, N. Adams, and P. Murray-Rust, "ChemicalTagger: A tool for semantic text-mining in chemistry," Journal of Cheminformatics, vol. 3, no. 7, 2011.
- M. Hearst, "What is Text Mining." [Online. Available: http://people.ischool.berkeley.edu/~hearst/text-mining.html [Accessed: 24-Nov-2011.
- The early work of Swanson was largely manual and later semi-automated in the Arrowsmith system [109]. While it is therefore different from what is now understood by text mining, Swanson is credited with having introduced a vision and a methodology (Swanson linking) that has helped the field evolve. For example, people will attempt to demonstrate how good their automated text mining systems are by attempting to replicate his early findings.
- Thomson Reuters, "Web of Knowledge," 2011. [Online. Available: http://wokinfo.com/ [Accessed: 07-Jan-2012.
- UKPMC, "UK PubMed Central." [Online. Available: http://ukpmc.ac.uk/About.
- I. Hargreaves, "Supporting Document Q: Fair use and the Independent Review," 2010.
- Open Access (OA) is a wide reaching phenomenon, which in the higher education context generally refers to unrestricted access to peer-reviewed scholarly articles. The OA movement, driven by the wish to make publicly-funded research results more readily available, increasingly includes access to grey publications such as technical reports and unpublished research.
- UKPMC, "UK PubMed Central." [Online. Available: http://ukpmc.ac.uk/About.
- J. Thomas and A. O'Mara-Eves, "Reconceptualising searching and screening: How new technologies might change the way that we identify studies," in 19th Cochran Colloquim, 2011.
- D. R. Swanson, "Fish oil, Raynaud's syndrome, and undiscovered public knowledge," Perspectives in Biology and Medicine, vol. 30, pp. 7-18, 1986.
- N. R. Smalheiser and D. R. Swanson, "Indomethacin and Alzheimer's disease," Neurology, no. 46, p. 583, 1996.
- S. Ananiadou, D. B. Kell, and J.-ichi Tsujii, "Text mining and its potential applications in systems biology.," Trends in biotechnology, vol. 24, no. 12, pp. 571-9, Dec. 2006.
- Stanford Humanities Center, "DIGGING INTO THE ENLIGHTENMENT." [Online. Available: http://web.archive.org/web/20131016094357/http://enlightenment.humanitie... [Accessed: 12-Feb-2012].
- J.-D. Kim, T. Ohta, K. Oda, and J. Tsujii, "From Text To Pathway: Corpus Annotation for Knowledge Acquisition From Biomedical Literature," Proceedings of The 6th Asia-Pacific Bioinformatics Conference, vol. 11, no. 1, pp. 165-175, 2008.
- S. Ananiadou, D. B. Kell, and J.-ichi Tsujii, "Text mining and its potential applications in systems biology.," Trends in biotechnology, vol. 24, no. 12, pp. 571-9, Dec. 2006.
- T. Ozasa, G. Weir, and M. Fukui, "A software application for assessing readability in the Japanese EFL context," Themes in Science and Technology Education, vol. 3, no. 1&2, pp. 91-118, 2010.
- G. R. S. Weir, S. Ishikawa, and K. Poonpon, Eds., Corpora and Language Technologies in Teaching, Learning and Research. University od Strathclyde Publishing, 2011.
- JISC, "Social media 'not to blame' for inciting rioters."[Online. Available: http://www.jisc.ac.uk/news/stories/2011/12/riot.aspx [Accessed: 21-Jan-2012.
- R. Rodriguez-Esteban, "Biomedical text mining and its applications.," PLoS computational biology, vol. 5, no. 12, p. e1000597, Dec. 2009.
- P. J. Herron, "Text Mining Adoption for Pharmacogenomics-based Drug Discovery in a Large Pharmaceutical Company: a Case Study," Library, 2006.
- H. Jung et al., "Semantic Web Use Cases and Case Studies Case Study?: iLaw — Intelligent Legislation Support System," Science And Technology, pp. 1-8, 2010.
- M. W. Berry and M. Castellanos, Survey of text mining II: clustering, classification, and retrieval, Volume 2. Springer, 2007.
- P. Thompson, "Text mining, names and security," Journal of Database management, vol. 6, no. 1, pp. 54-59, 2005.
- It should be noted that there is a degree of overlap in the categories listed below. However, this categorisation was chosen as it proved a useful framework in which to analyse the costs, benefits and barriers to text mining.
- The core part of this post is concerned with obtaining permission although some other activities are also included.
- University of Nottingham, "SHERPA/RoMeO: Publisher copyright policies & self-archiving," 2011. [Online. Available: http://www.sherpa.ac.uk/romeo [Accessed: 12-Feb-2012.
- Stanford Humanities Center, "DIGGING INTO THE ENLIGHTENMENT." [Online. Available: http://web.archive.org/web/20131016094357/http://enlightenment.humanitie... [Accessed: 12-Feb-2012.
- "Peer Review Workshop on the Value and benefits of text Mining to UKFHE, 10/02/12." 2012.
- J. Manyika et al., "Big data?: The next frontier for innovation , competition , and productivity," 2011.
- Collections could consist of scholarly articles or research data such as policy documents, social media discussions etc.
- Institute of Education, "The UK Educational Evidence Portal," 2009. [Online. Available: http://www.eep.ac.uk/dnn2/tabid/36/Default.aspx [Accessed: 12-Feb-2012.
- S. Ananiadou et al., "Supporting the education evidence portal via text mining.," Philosophical transactions. Series A, Mathematical, physical, and engineering sciences, vol. 368, no. 1925, pp. 3829-44, Aug. 2010.
- J. Thomas and A. O'Mara-Eves, "Reconceptualising searching and screening: How new technologies might change the way that we identify studies," in 19th Cochran Colloquim, 2011.
- M. Weeber, R. Vos, H. Klein, L. T. W. de Jong-Van den Berg, A. A., and G. Molema, "Generating hypotheses by discovering implicit associations in the literature: A case report for new potential therapeutic uses for Thalidomide," Journal of the American Medical Informatics Association, vol. 10, no. 3, pp. 252-259, 2003.
- Institute of Education, "The UK Educational Evidence Portal," 2009. [Online. Available: http://www.eep.ac.uk/dnn2/tabid/36/Default.aspx [Accessed: 12-Feb-2012.
- EMBL-EBI, "ChEBI." [Online. Available: http://www.ebi.ac.uk/chebi [Accessed: 12-Feb-2012.
- Nanopub.org, "nanopub: a beginner's guide to datapublishing." [Online. Accessed: 15-Feb-2012.]
- University of Manchester, "University enters collaboration to develop text mining applications," 2011. [Online. Available: http://www.manchester.ac.uk/aboutus/news/display/?id=7627.
- "Mendeley," Mendeley. [Online. Available: http://www.mendeley.com [Accessed: 30-Nov-2011.
- Office of Digital Humanities, "The Digging into Data Challenge," 2012. [Online. Available: http://www.diggingintodata.org/ [Accessed: 21-Jan-2012.
- JISC, "Data Mining with Criminal Intent," 2011. [Online. Available: http://www.jisc.ac.uk/whatwedo/programmes/digitisation/diggingintodata/c....
- D. McDonald, E. McCulloch, A. MacDonald, and JISC, "Greening Information Management: Final report." University of Strathclyde, pp. 1-42, 2009.
- University of Strathclyde, "Review of the Environmental and Organisational Implications of Cloud Computing in Higher and Further Education," no. 13/05/10.
- BioMed Central, Available: http://www.biomedcentral.com/
- British Library, "Response from the British Library to the Independent Review of Intellectual Property and Growth .," 2011.
- Office of Digital Humanities, "The Digging into Data Challenge," 2012. [Online. Available: http://www.diggingintodata.org/ [Accessed: 21-Jan-2012.
- Documents where the copyright holder cannot be traced.
- HEFCE, "Research Excellence Framework," no. 01/09/09. 2009.
- H. Jung et al., "Semantic Web Use Cases and Case Studies Case Study?: iLaw — Intelligent Legislation Support System," Science And Technology, pp. 1-8, 2010.
- P. J. Herron, "Text Mining Adoption for Pharmacogenomics-based Drug Discovery in a Large Pharmaceutical Company: a Case Study," Library, 2006.
- "Peer Review Workshop on the Value and benefits of text Mining to UKFHE, 10/02/12." 2012.
- R. K. Yin, Case study research?: design and methods / Robert K. Yin. SAGE, 2009.
- C. Breslin and D. McDonald, "BIILS: Benefits of ICT investment landscape study phase 2," 2010.
- D. B. Kell, "Iron behaving badly: inappropriate iron chelation as a major contributor to the aetiology of vascular and other progressive inflammatory and degenerative diseases," BMC medical genomics, vol. 2, p. 2, Jan. 2009.
- D. B. Kell, "Iron behaving badly: inappropriate iron chelation as a major contributor to the aetiology of vascular and other progressive inflammatory and degenerative diseases," BMC medical genomics, vol. 2, p. 2, Jan. 2009.
- NaCTeM, "Kleio," 2010. [Online. Accessed: 10-Jan-2012.]
- NaCTeM, "Facta," 2010. [Online]. Available: http://web.archive.org/web/20130923030500/http://refine1-nactem.mc.man.a... [Accessed: 14-Jun-2015].
- Thomson Reuters, "Web of Knowledge," 2011. [Online. Available: http://wokinfo.com/ [Accessed: 07-Jan-2012.
- Elsevier, "Scopus," 2012. [Online. Available: http://www.scopus.com [Accessed: 10-Jan-2012.]
- Google, "Google scholar," 2011. [Online. Available: https://scholar.google.co.uk/ [Accessed: 10-Jan-2012.]
- CIBER, "EVALUATING THE USAGE E-JOURNALS IN THE UK UPON BREADTH OF CITATION??," no. November. pp. 1-27, 2008.
- Some references relate to text mining or information retrieval etc and not iron.
- Automatically generated summaries contain significant information that article abstracts do not. For example, Blake [110] calculated that, on average, abstracts contain fewer than 7.84% of scientific claims made in the full text articles.
- C. Tenopir, D. W. King, S. Edwards, and L. Wu, "Electronic journals and changes in scholarly article seeking and reading patterns," Aslib Proceedings, vol. 61, no. 1, pp. 5-32, 2009.
- Based on Tenopir and King's [70] longitudinal study of US academics' reading practices.
- C. Tenopir, D. W. King, S. Edwards, and L. Wu, "Electronic journals and changes in scholarly article seeking and reading patterns," Aslib Proceedings, vol. 61, no. 1, pp. 5-32, 2009.
- "JISC Text Mining Workshop 21st October." London, 2011.
- Automated summarising is undertaken once as part of the initial compilation of the text mining repository and is part of the initial entry costs. These summaries can then be accessed as required. The time for automated summarising is therefore not relevant in this calculation.
- ConnectedDiscovery, "ConnectedDiscovery: Enabling precompetitive knowledge management in the life sciences," 2012. [Online. Accessed: 21-Jan-2012.]
- The Pistoia Alliance, "SESL," 2012. [Online. Available: http://web.archive.org/web/20120712011655/http://www.pistoiaalliance.org... [Accessed: 21-Jan-2012.
- UKPMC, "UK PubMed Central." [Online]. Available: http://ukpmc.ac.uk/About
- This search was conducted on 1 November 2011 by staff at the European Bioinformatics Institute. A copy of the spread-sheet listing those journals that appeared in the non OA cohort is available on request.
- Typically around 35% of the content in UKPMC is OA. The exceptionally high OA figure for malaria is a reflection of the fact that the Wellcome Trust is a key funder of malaria research, and it requires all the research it funds to be made Open Access.
- The British Library estimated that it took 302 hours just to identify 299 rights holders.
- This calculation assumes that there are 220 working days in a year, and that a researcher works 7.5 hours a day. This equates to 1,650 hours a year, of which 187 hours equals 11.3%. Starting salary for newly qualified researchers based on UCL data, available at: http://www.ucl.ac.uk/hr/salary_scales/final_grades.php
- The number of research articles in UKPMC, published between 2000 and 2011, which contain the term 'malaria' totals 15,757. Of these, 7,998 articles (published in 557 journals), were Open Access, whilst the remaining articles (7759, published in 1024 journals) were not Open Access. This analysis was conducted on 22 December 2011 by the Wellcome Trust. The dataset is available on request.
- JISC/MIMAS, "JISC Journal Archives." [Online. Available: http://www.jiscjournalarchives.ac.uk/index.html [Accessed 21-Jan-2012.
- Individual institutions are required to subscribe to the service.
- C. Tenopir, D. W. King, S. Edwards, and L. Wu, "Electronic journals and changes in scholarly article seeking and reading patterns," Aslib Proceedings, vol. 61, no. 1, pp. 5-32, 2009.
- The Pistoia Alliance, "SESL," 2012. [Online. Available: http://web.archive.org/web/20120712011655/http://www.pistoiaalliance.org... [Accessed: 21-Jan-2012.
- £416,168 x 144,000 = £59,927,040
- (31 minutes manual reading)/(5 minutes to read summary)
- M. Hucka et al., "Evolving a Lingua Franca and Associated Software Infrastructure for Computational Systems Biology - the Systems Biology Markup Language (SBML) Project.," Systems Biology, vol. 1, no. 1, pp. 41-53, 2004.
- J.-D. Kim, T. Ohta, K. Oda, and J. Tsujii, "From Text To Pathway: Corpus Annotation for Knowledge Acquisition From Biomedical Literature," Proceedings of The 6th Asia-Pacific Bioinformatics Conference, vol. 11, no. 1, pp. 165-175, 2008.
- E. Donnard et al., "Preimplantation development regulatory pathway construction through a text-mining approach," BMC Genomics, vol. 12, no. 4, p. S3, 2011.
- EMBL-EBI, "ChEBI." [Online. Available: http://www.ebi.ac.uk/chebi [Accessed: 12-Feb-2012.
- ChEBI has five curators.
- "Peer Review Workshop on the Value and benefits of text Mining to UKFHE, 10/02/12." 2012.
- B. Alex et al., "Assisted curation: does text mining really help?," Pacific Symposium on Biocomputing. Pacific Symposium on Biocomputing, vol. 567, pp. 556-67, Jan. 2008.
- It should be noted that the Alex, Grover et al [77] was a relatively small study; however, it is indicative of the types of efficiency savings that could be accrued.
- B. Alex et al., "Assisted curation: does text mining really help?," Pacific Symposium on Biocomputing. Pacific Symposium on Biocomputing, vol. 567, pp. 556-67, Jan. 2008.
- B. Alex et al., "Assisted curation: does text mining really help?," Pacific Symposium on Biocomputing. Pacific Symposium on Biocomputing, vol. 567, pp. 556-67, Jan. 2008.
- Alex, Grover at al [77] figures also include figures for text mining enhanced curation without curator assistance. These are omitted here for clarity as the curator assisted curation was the most efficient. See Alex, Grover at al [77] for full details of the experiment and their findings.
- Based on a curator as a non-academic professional with average salary of £36,040 (mid of salary scale £30,747 – £41,333) and 220 working days pa, 7.5 hours per day.
- "Mendeley," Mendeley. [Online. Available: http://www.mendeley.com [Accessed: 30-Nov-2011.
- "Start-Up of the Year" at the 2009 Plugg Conference; "Best Social Innovation Which Benefits Society" at the 2009 TechCrunch Europe Awards; "Start-Up Most Likely To Change The World For The Better" at the 2010 Guardian Activate Summit; "Best Education Start-Up" at the 2011 Telegraph 100; Microsoft/Sunday Times "Tech Track 100" Award 2011
- Not all publishers allow Mendeley to provide two page abstracts of their articles.
- Negotiations are underway with several others
- Mendeley, "Announcing the Mendeley Institutional Edition – powered by Swets," 2011. [Online. Available: http://web.archive.org/web/20120206062735/http://www.mendeley.com/blog/a... [Accessed: 14-Jun-2015.
- Mendeley, "Winners of the first Binary Battle Apps for Science Contest," 2011. [Online. Available: http://web.archive.org/web/20120211000447/http://www.mendeley.com/blog/d... [Accessed: 2015.
- I. Hargreaves, "Digital Opportunity: A Review of Intellectual Property and Growth," 2011.
- From the consultations it appeared that there is a larger body of 'text mining researchers' focused on the development of, and experimentation with, text mining tools, with a much smaller body of 'researchers using text mining' (ieusing text mining simply as another tool). Much of the UKFHE text mining tool development has focused on tools for biomedical uses – possibly, in part at least, as a natural result of the legal restrictions on material that can be text mined – since UK PubMed Central, as an Open Access repository, is one of the few current sources of material that can be legally text mined without seeking additional explicit permission.
- D. Delen and M. D. Crossland, "Seeding the survey and analysis of research literature with text mining," Expert Systems with Applications, vol. 34, no. 3, pp. 1707-1720, Apr. 2008.
- N. Harmston, W. Filsell, and M. P. H. Stumpf, "What the papers say: text mining for genomics and systems biology.," Human genomics, vol. 5, no. 1, pp. 17-29, Oct. 2010.
- U. Kelly, D. McLellan, and I. McNicoll, "The impact of universities on the UK economy," Journal of Policy Research in Tourism Leisure and Events, pp. 1-28, 2009.
- Comparable figures are not available for the FE sector across the UK, although a 2007 study of FE in England showed full-time equivalent employment of over 170,000 people and turnover of nearly £7bn
- Higher Education Statistics Agency, "Staff in Higher Education Institutions 2009/10," 2011.
- The 'headcount' equivalent was 181,595 people.
- We are basing our calculations on UK HESA data which gives a more conservative figure for the cost of researcher time than that used by CEPA. We are using Research Council norms for number of working hours per year (1,650 hours).
- This equates to 33 hours per full time equivalent academic per year (220 days and 44 working weeks per year).
- CIBER, "EVALUATING THE USAGE E-JOURNALS IN THE UK UPON BREADTH OF CITATION??," no. November. pp. 1-27, 2008.
- In collaboration with the Publishing Research Consortium, the Society of College, National and University Libraries (SCONUL) and Research Libraries UK (RLUK).
- Cambridge Economic Policy Associates, "Activities, costs and funding flows in the scholarly communications system in the UK Report commissioned by the Research Information Network ( RIN ) Full Report," 2008.
- It excluded other forms of scholarly outputs e.g. monographs, conference proceedings etc.
- Cambridge Economic Policy Associates, "Activities, costs and funding flows in the scholarly communications system in the UK: Summary Report."
- Cambridge Economic Policy Associates, "Activities, costs and funding flows in the scholarly communications system?: Key findings," 2008.
- C. Tenopir, D. W. King, S. Edwards, and L. Wu, "Electronic journals and changes in scholarly article seeking and reading patterns," Aslib Proceedings, vol. 61, no. 1, pp. 5-32, 2009.
- Of these 280 articles, 204 are unique.
- C. Tenopir, D. W. King, S. Edwards, and L. Wu, "Electronic journals and changes in scholarly article seeking and reading patterns," Aslib Proceedings, vol. 61, no. 1, pp. 5-32, 2009.
- Department for Business Innovation and Skills, "Innovation and Research Strategy for Growth," 2011.
- Further, a search for literature on the economic impact of copyright, market failure and related issues revealed very little hard or empirical evidence on the impact of copyright or on how copyright supported innovation – this evidence gap which was also noted in the BIS economic report
- European Commission, "INNOVATION UNION SCOREBOARD 2010," 2011.
- K. Allman et al., "Measuring Wider Framework Conditions for successful innovation: A system's review of UK and international innovation data," 2011.
- N. Miles, C. Wilkinson, J. Edler, M. Bleda, P. Simmonds, and J. Clark, "The wider conditions for innovation in the UK: How the UK compares to leading innovation nations," 2009.
- Higher Education Statistics Agency, "Finances of Higher Education Institutes 2009-2010." 2011.
- Department for Business Innovation and Skills, "Innovation and Research Strategy for Growth," 2011.
- Department for Business Innovation and Skills, "Innovation and Research Strategy for Growth," 2011.
- Department for Business Innovation and Skills, "Innovation and Research Strategy for Growth," 2011.
- H. Chesbrough, Open Innovation: The New Imperative for Creating and Profiting from Technology . Boston: Harvard Business School Press, 2003.
- A. L. Porter and N. C. Newman, "Mining external R&D," Technovation, vol. 31, no. 4, pp. 171-176, Apr. 2011.
- A. L. Porter and N. C. Newman, "Mining external R&D," Technovation, vol. 31, no. 4, pp. 171-176, Apr. 2011.
- Department for Business Innovation and Skills, "BIS Economics Paper No.15 Innovation and Research Strategy for Growth," no. 15. 2011.
- Department for Business Innovation and Skills, "BIS Economics Paper No.15 Innovation and Research Strategy for Growth," no. 15. 2011.
- E. Smit and M. Van der Graaf, "Journal article mining," 2011. Available: http://web.archive.org/web/20140102230201/http://publishingresearch.net/ [Accessed 13-Mar-2012
- Further, a search for literature on the economic impact of copyright, market failure and related issues revealed very little hard or empirical evidence on the impact of copyright or on how copyright supported innovation – this evidence gap which was also noted in the BIS economic report
- Welfare economics is a 'branch of economics that focuses on the optimal allocation of resources and goods and how this affects social welfare. Welfare economics analyses the total good or welfare that is achieved at a current state as well as how it is distributed'
- It is beyond the resources and the scope of this study to discuss the economic theory and its practical application in detail
- HM Treasury, The Green Book: Appraisal and Evaluation in Central Government, no. July. 2011, pp. 57-58.
- The 'Green Book' is a best practice guide in project and programme appraisal for government central departments and executive agencies. It draws on fundamental economic theory to give recommendations and guidance on economic, financial, social and environmental assessments.
- This is about the most effective use of resources to get the best overall outcome for society as a whole. An economy could be 'economically efficient' and still have disparities of wealth between citizens. It could mean for example that the rich get richer but as long as this does not mean that the poor get poorer this would be economically efficient.
- M. E. Porter, "Location, Competition, and Economic Development: Local Clusters in a Global Economy," Economic Development Quarterly, vol. 14, no. 1, pp. 15-34, Feb. 2000.
- M. Delgado, M. E. Porter, and S. Stern, "Clusters and entrepreneurship," Journal of Economic Geography, vol. 10, no. 4, pp. 495-518, May 2010.
- Knowledge spillovers are also part of theories of innovation
- E. Smit and M. Van der Graaf, "Journal article mining," 2011. Available: http://web.archive.org/web/20140102230201/http://publishingresearch.net/ [Accessed 13-Mar-2012
- The recent study into Journal Article Mining [8] indicated that 70% of the publishers surveyed required information from prospective users about the 'intent and purpose' of the mining request so that they could decide whether or not to grant permission.
- I. Hargreaves, "Digital Opportunity: A Review of Intellectual Property and Growth," 2011.
- British Library, "Response from the British Library to the Independent Review of Intellectual Property and Growth .," 2011.
- E. Smit and M. Van der Graaf, "Journal article mining," 2011. Available: http://web.archive.org/web/20140102230201/http://publishingresearch.net/ [Accessed 13-Mar-2012
- Department for Business Innovation and Skills, "BIS Economics Paper No.15 Innovation and Research Strategy for Growth," no. 15. 2011.
- R. Corrigan and M. Rogers, "The Economics of Copyright," English, vol. 6, no. 3, pp. 153-174, 2005.
- M. Rogers, J. Tomalin, and R. Corrigan, "The economic impact of consumer copyright exceptions?: A literature review."
- M. Ware and M. Mabe, "The stm report: An overview of scientific and scholarly journal publishing," 2009.
- Cambridge Economic Policy Associates, "Activities, costs and funding flows in the scholarly communications system in the UK Report commissioned by the Research Information Network ( RIN ) Full Report," 2008.
- The 'Innovation and Research Strategy for Growth' is BIS economics paper No. 15 http://bis.gov.uk/innovatingforgrowth
- Department for Business Innovation and Skills, "Innovation and Research Strategy for Growth," 2011.
- Department for Business Innovation and Skills, "Innovation and Research Strategy for Growth," 2011.
- Pricewaterhouse Coopers, "An economic analysis of copyright, secondary copyright and collective licensing," 2011.
- Cambridge Economic Policy Associates, "Activities, costs and funding flows in the scholarly communications system in the UK Report commissioned by the Research Information Network ( RIN ) Full Report," 2008.
- M. Ware and M. Mabe, "The stm report: An overview of scientific and scholarly journal publishing," 2009.
- I. Hargreaves, "Digital Opportunity: A Review of Intellectual Property and Growth," 2011.
- HM Treasury, The Green Book: Appraisal and Evaluation in Central Government, no. July. 2011, pp. 57-58.
- J. Manyika et al., "Big data?: The next frontier for innovation , competition , and productivity," 2011.
- Facebook users share over 30 million pieces of content per month [3] and twitter has 350 million tweets daily
- J. Manyika et al., "Big data : The next frontier for innovation , competition , and productivity," 2011.
- The MGI study focuses specifically on what it terms 'Big Data' datasets whose size is beyond the ability of typical database software tools to capture, store, manage, and analyse
- MoneyWeek, "How Tesco became Britain's top supermarket," 2007. [Online. Accessed: 15-Feb-2012.]
- S. Grimes, "Text-Analytics Demand Approaches $1 Billion," InformationWeek, 2011. [Online. Accessed: 30-Nov-2011.]
- ScraperWiki, "ScraperWiki." [Online. Available: https://scraperwiki.com/ [Accessed: 30-Nov-2011.
- CommonCrawl, "CommonCrawl." [Online. Available: http://www.commoncrawl.org/ [Accessed: 30-Nov-2011.
- E. Smit and M. Van der Graaf, "Journal article mining," 2011. Available: http://web.archive.org/web/20140102230201/http://publishingresearch.net/ [Accessed 13-Mar-2012
- N. R. Smalheiser and D. R. Swanson, "Indomethacin and Alzheimer's disease," Neurology, no. 46, p. 583, 1996.
- Gartner Inc., "Gartner Identifies the Top 10 Strategic Technologies for 2012," 2011. [Online.]
- I. Hargreaves, "Digital Opportunity: A Review of Intellectual Property and Growth," 2011.
- Compare www.cs.cmu.edu/~dunja/CFPWshKDD2000.html with http://web.archive.org/web/20120303042253/http://www.ir.iit.edu/cikm2004...
- UKPMC, "UK PubMed Central." [Online. Available: http://ukpmc.ac.uk/About.
- Institute of Education, "The UK Educational Evidence Portal," 2009. [Online. Available: http://www.eep.ac.uk/dnn2/tabid/36/Default.aspx [Accessed: 12-Feb-2012.
- Office of Digital Humanities, "The Digging into Data Challenge," 2012. [Online. Available: http://www.diggingintodata.org/ [Accessed: 21-Jan-2012.
- S. Ananiadou, J. Chruszcz, J. Keane, J. McNaught, and P. Watry, "The National Centre for Text Mining: Aims and Objectives," Ariadne, no. 42, 2005.
- K. Allman et al., "Measuring Wider Framework Conditions for successful innovation: A system's review of UK and international innovation data," 2011.
- U. Kelly, D. McLellan, and I. McNicoll, "The Impact of Universities on the UK Economy," 2009.
- In.Dig.O, "Analysis of the Value and Benefits of Text Mining and Text Analytics to UK HE and FE." [Online. Accessed: 21-Jan-2012.]
- I. Hargreaves, "Digital Opportunity: A Review of Intellectual Property and Growth," 2011.
- HM Treasury, The Green Book: Appraisal and Evaluation in Central Government, no. July. 2011, pp. 57-58.
- P. A. Champ, K. J. Boyle, and T. C. (Eds. . Brown, A Primer on Nonmarket Valuation. Kluwer Academic Publishers, 2003.
- HM Treasury, The Green Book: Appraisal and Evaluation in Central Government, no. July. 2011, pp. 57-58.
- A fifth stage – exploring exploitation (barriers, solutions and resources) – had been planned; however, this was rolled into the consultation and case study phases. Further details of the study approach can be found on the project website
- While anonymised case studies were considered to encourage participation, it was clear that it would be difficult then to meet the IPO's evidence criteria
- HM Treasury, The Green Book: Appraisal and Evaluation in Central Government, no. July. 2011, pp. 57-58.
- HM Treasury, The Magenta Book: Guidance for evaluation. 2011.
- HM Treasury, "The Orange Book: Management of Risk- Principles and Concepts," 2004.
- Intellectual Property Office, "Good Evidence for Policy," 2012. [Online. Available: http://webarchive.nationalarchives.gov.uk/20140603093549/http://www.ipo.... [Accessed: 16-Jan-2012.
- Office of Digital Humanities, "The Digging into Data Challenge," 2012. [Online. Available: http://www.diggingintodata.org/ [Accessed: 21-Jan-2012.
- HathiTrust Research Center, "HathiTrust Research Center." [Online. Available: http://www.hathitrust.org/htrc [Accessed: 15-Feb-2012.
- Eigenfactor.org, "Eigenfactor.org." [Online. Available: http://www.eigenfactor.org/about.php [Accessed: 15-Feb-2012.
- T. J. Larsson, M. Jansson, and B. Brooks, "TEXT-MINING OF INSURANCE-BASED INFORMATION: DECISION SUPPORT FOR LOCAL SAFETY MANAGEMENT," Safety Science Monitor, no. 1, pp. 1-9, 2009.
- University of Tokyo, "Tsujii Laboratory." [Online. Available: http://web.archive.org/web/20140722105856/http://www.nactem.ac.uk/tsujii/ [Accessed: 15-Feb-2012.
- MacQuarie University, "Centre for Language Technology." [Online. Available: http://clt.mq.edu.au/ [Accessed: 15-Feb-2012.
- Ben-Gurion University, "Text Mining Day 2009." [Online. Available: http://www.cs.bgu.ac.il/~frankel/TextMiningMay09/abstracts_bios.html [Accessed: 15-Feb-2012.
- Fraunhofer AIAS, "Text Mining and Information Retrieval." [Online. Available: http://web.archive.org/web/20130117005356/http://www.iais.fraunhofer.de/... [Accessed: 15-Feb-2012.
- Chinese Academy of Sciences, "Chemical Informatics Improved Text Mining." [Online. Available: http://web.archive.org/web/20121011070350/http://english.cas.cn/Ne/ICN/2... [Accessed: 03-Feb-2015.
- M. Ware and M. Mabe, "The STM Report: An overview of scientific and scholarly journal publishing," 2009.
- A broad key-word search could potentially bring more journals to the attention of the researcher than (s)he would have looked at manually.
- L. Hawizy, D. M. Jessop, N. Adams, and P. Murray-Rust, "ChemicalTagger: A tool for semantic text-mining in chemistry," Journal of Cheminformatics, vol. 3, no. 7, 2011.
- M. Hearst, "What is Text Mining." [Online. Available: http://people.ischool.berkeley.edu/~hearst/text-mining.html [Accessed: 24-Nov-2011.
- The early work of Swanson was largely manual and later semi-automated in the Arrowsmith system [109]. While it is therefore different from what is now understood by text mining, Swanson is credited with having introduced a vision and a methodology (Swanson linking) that has helped the field evolve. For example, people will attempt to demonstrate how good their automated text mining systems are by attempting to replicate his early findings.
- Thomson Reuters, "Web of Knowledge," 2011. [Online. Available: http://wokinfo.com/ [Accessed: 07-Jan-2012.
- UKPMC, "UK PubMed Central." [Online. Available: http://ukpmc.ac.uk/About.
- I. Hargreaves, "Supporting Document Q: Fair use and the Independent Review," 2010.
- Open Access (OA) is a wide reaching phenomenon, which in the higher education context generally refers to unrestricted access to peer-reviewed scholarly articles. The OA movement, driven by the wish to make publicly-funded research results more readily available, increasingly includes access to grey publications such as technical reports and unpublished research.
- UKPMC, "UK PubMed Central." [Online. Available: http://ukpmc.ac.uk/About.
- J. Thomas and A. O'Mara-Eves, "Reconceptualising searching and screening: How new technologies might change the way that we identify studies," in 19th Cochran Colloquim, 2011.
- D. R. Swanson, "Fish oil, Raynaud's syndrome, and undiscovered public knowledge," Perspectives in Biology and Medicine, vol. 30, pp. 7-18, 1986.
- N. R. Smalheiser and D. R. Swanson, "Indomethacin and Alzheimer's disease," Neurology, no. 46, p. 583, 1996.
- S. Ananiadou, D. B. Kell, and J.-ichi Tsujii, "Text mining and its potential applications in systems biology.," Trends in biotechnology, vol. 24, no. 12, pp. 571-9, Dec. 2006.
- Stanford Humanities Center, "DIGGING INTO THE ENLIGHTENMENT." [Online. Available: http://web.archive.org/web/20131016094357/http://enlightenment.humanitie... [Accessed: 12-Feb-2012].
- J.-D. Kim, T. Ohta, K. Oda, and J. Tsujii, "From Text To Pathway: Corpus Annotation for Knowledge Acquisition From Biomedical Literature," Proceedings of The 6th Asia-Pacific Bioinformatics Conference, vol. 11, no. 1, pp. 165-175, 2008.
- S. Ananiadou, D. B. Kell, and J.-ichi Tsujii, "Text mining and its potential applications in systems biology.," Trends in biotechnology, vol. 24, no. 12, pp. 571-9, Dec. 2006.
- T. Ozasa, G. Weir, and M. Fukui, "A software application for assessing readability in the Japanese EFL context," Themes in Science and Technology Education, vol. 3, no. 1&2, pp. 91-118, 2010.
- G. R. S. Weir, S. Ishikawa, and K. Poonpon, Eds., Corpora and Language Technologies in Teaching, Learning and Research. University od Strathclyde Publishing, 2011.
- JISC, "Social media 'not to blame' for inciting rioters."[Online. Available: http://www.jisc.ac.uk/news/stories/2011/12/riot.aspx [Accessed: 21-Jan-2012.
- R. Rodriguez-Esteban, "Biomedical text mining and its applications.," PLoS computational biology, vol. 5, no. 12, p. e1000597, Dec. 2009.
- P. J. Herron, "Text Mining Adoption for Pharmacogenomics-based Drug Discovery in a Large Pharmaceutical Company: a Case Study," Library, 2006.
- H. Jung et al., "Semantic Web Use Cases and Case Studies Case Study?: iLaw — Intelligent Legislation Support System," Science And Technology, pp. 1-8, 2010.
- M. W. Berry and M. Castellanos, Survey of text mining II: clustering, classification, and retrieval, Volume 2. Springer, 2007.
- P. Thompson, "Text mining, names and security," Journal of Database management, vol. 6, no. 1, pp. 54-59, 2005.
- It should be noted that there is a degree of overlap in the categories listed below. However, this categorisation was chosen as it proved a useful framework in which to analyse the costs, benefits and barriers to text mining.
- The core part of this post is concerned with obtaining permission although some other activities are also included.
- University of Nottingham, "SHERPA/RoMeO: Publisher copyright policies & self-archiving," 2011. [Online. Available: http://www.sherpa.ac.uk/romeo [Accessed: 12-Feb-2012.
- Stanford Humanities Center, "DIGGING INTO THE ENLIGHTENMENT." [Online. Available: http://web.archive.org/web/20131016094357/http://enlightenment.humanitie... [Accessed: 12-Feb-2012.
- "Peer Review Workshop on the Value and benefits of text Mining to UKFHE, 10/02/12." 2012.
- J. Manyika et al., "Big data?: The next frontier for innovation , competition , and productivity," 2011.
- Collections could consist of scholarly articles or research data such as policy documents, social media discussions etc.
- Institute of Education, "The UK Educational Evidence Portal," 2009. [Online. Available: http://www.eep.ac.uk/dnn2/tabid/36/Default.aspx [Accessed: 12-Feb-2012.
- S. Ananiadou et al., "Supporting the education evidence portal via text mining.," Philosophical transactions. Series A, Mathematical, physical, and engineering sciences, vol. 368, no. 1925, pp. 3829-44, Aug. 2010.
- J. Thomas and A. O'Mara-Eves, "Reconceptualising searching and screening: How new technologies might change the way that we identify studies," in 19th Cochran Colloquim, 2011.
- M. Weeber, R. Vos, H. Klein, L. T. W. de Jong-Van den Berg, A. A., and G. Molema, "Generating hypotheses by discovering implicit associations in the literature: A case report for new potential therapeutic uses for Thalidomide," Journal of the American Medical Informatics Association, vol. 10, no. 3, pp. 252-259, 2003.
- Institute of Education, "The UK Educational Evidence Portal," 2009. [Online. Available: http://www.eep.ac.uk/dnn2/tabid/36/Default.aspx [Accessed: 12-Feb-2012.
- EMBL-EBI, "ChEBI." [Online. Available: http://www.ebi.ac.uk/chebi [Accessed: 12-Feb-2012.
- Nanopub.org, "nanopub: a beginner's guide to datapublishing." [Online. Accessed: 15-Feb-2012.]
- University of Manchester, "University enters collaboration to develop text mining applications," 2011. [Online. Available: http://www.manchester.ac.uk/aboutus/news/display/?id=7627.
- "Mendeley," Mendeley. [Online. Available: http://www.mendeley.com [Accessed: 30-Nov-2011.
- Office of Digital Humanities, "The Digging into Data Challenge," 2012. [Online. Available: http://www.diggingintodata.org/ [Accessed: 21-Jan-2012.
- JISC, "Data Mining with Criminal Intent," 2011. [Online. Available: http://www.jisc.ac.uk/whatwedo/programmes/digitisation/diggingintodata/c....
- D. McDonald, E. McCulloch, A. MacDonald, and JISC, "Greening Information Management: Final report." University of Strathclyde, pp. 1-42, 2009.
- University of Strathclyde, "Review of the Environmental and Organisational Implications of Cloud Computing in Higher and Further Education," no. 13/05/10.
- BioMed Central, Available: http://www.biomedcentral.com/
- British Library, "Response from the British Library to the Independent Review of Intellectual Property and Growth .," 2011.
- Office of Digital Humanities, "The Digging into Data Challenge," 2012. [Online. Available: http://www.diggingintodata.org/ [Accessed: 21-Jan-2012.
- Documents where the copyright holder cannot be traced.
- HEFCE, "Research Excellence Framework," no. 01/09/09. 2009.
- H. Jung et al., "Semantic Web Use Cases and Case Studies Case Study?: iLaw — Intelligent Legislation Support System," Science And Technology, pp. 1-8, 2010.
- P. J. Herron, "Text Mining Adoption for Pharmacogenomics-based Drug Discovery in a Large Pharmaceutical Company: a Case Study," Library, 2006.
- "Peer Review Workshop on the Value and benefits of text Mining to UKFHE, 10/02/12." 2012.
- R. K. Yin, Case study research?: design and methods / Robert K. Yin. SAGE, 2009.
- C. Breslin and D. McDonald, "BIILS: Benefits of ICT investment landscape study phase 2," 2010.
- D. B. Kell, "Iron behaving badly: inappropriate iron chelation as a major contributor to the aetiology of vascular and other progressive inflammatory and degenerative diseases," BMC medical genomics, vol. 2, p. 2, Jan. 2009.
- D. B. Kell, "Iron behaving badly: inappropriate iron chelation as a major contributor to the aetiology of vascular and other progressive inflammatory and degenerative diseases," BMC medical genomics, vol. 2, p. 2, Jan. 2009.
- NaCTeM, "Kleio," 2010. [Online. Accessed: 10-Jan-2012.]
- NaCTeM, "Facta," 2010. [Online]. Available: http://web.archive.org/web/20130923030500/http://refine1-nactem.mc.man.a... [Accessed: 14-Jun-2015].
- Thomson Reuters, "Web of Knowledge," 2011. [Online. Available: http://wokinfo.com/ [Accessed: 07-Jan-2012.
- Elsevier, "Scopus," 2012. [Online. Available: http://www.scopus.com [Accessed: 10-Jan-2012.]
- Google, "Google scholar," 2011. [Online. Available: https://scholar.google.co.uk/ [Accessed: 10-Jan-2012.]
- CIBER, "EVALUATING THE USAGE E-JOURNALS IN THE UK UPON BREADTH OF CITATION??," no. November. pp. 1-27, 2008.
- Some references relate to text mining or information retrieval etc and not iron.
- Automatically generated summaries contain significant information that article abstracts do not. For example, Blake [110] calculated that, on average, abstracts contain fewer than 7.84% of scientific claims made in the full text articles.
- C. Tenopir, D. W. King, S. Edwards, and L. Wu, "Electronic journals and changes in scholarly article seeking and reading patterns," Aslib Proceedings, vol. 61, no. 1, pp. 5-32, 2009.
- Based on Tenopir and King's [70] longitudinal study of US academics' reading practices.
- C. Tenopir, D. W. King, S. Edwards, and L. Wu, "Electronic journals and changes in scholarly article seeking and reading patterns," Aslib Proceedings, vol. 61, no. 1, pp. 5-32, 2009.
- "JISC Text Mining Workshop 21st October." London, 2011.
- Automated summarising is undertaken once as part of the initial compilation of the text mining repository and is part of the initial entry costs. These summaries can then be accessed as required. The time for automated summarising is therefore not relevant in this calculation.
- ConnectedDiscovery, "ConnectedDiscovery: Enabling precompetitive knowledge management in the life sciences," 2012. [Online. Accessed: 21-Jan-2012.]
- The Pistoia Alliance, "SESL," 2012. [Online. Available: http://web.archive.org/web/20120712011655/http://www.pistoiaalliance.org... [Accessed: 21-Jan-2012.
- UKPMC, "UK PubMed Central." [Online]. Available: http://ukpmc.ac.uk/About
- This search was conducted on 1 November 2011 by staff at the European Bioinformatics Institute. A copy of the spread-sheet listing those journals that appeared in the non OA cohort is available on request.
- Typically around 35% of the content in UKPMC is OA. The exceptionally high OA figure for malaria is a reflection of the fact that the Wellcome Trust is a key funder of malaria research, and it requires all the research it funds to be made Open Access.
- The British Library estimated that it took 302 hours just to identify 299 rights holders.
- This calculation assumes that there are 220 working days in a year, and that a researcher works 7.5 hours a day. This equates to 1,650 hours a year, of which 187 hours equals 11.3%. Starting salary for newly qualified researchers based on UCL data, available at: http://www.ucl.ac.uk/hr/salary_scales/final_grades.php
- The number of research articles in UKPMC, published between 2000 and 2011, which contain the term 'malaria' totals 15,757. Of these, 7,998 articles (published in 557 journals), were Open Access, whilst the remaining articles (7759, published in 1024 journals) were not Open Access. This analysis was conducted on 22 December 2011 by the Wellcome Trust. The dataset is available on request.
- JISC/MIMAS, "JISC Journal Archives." [Online. Available: http://www.jiscjournalarchives.ac.uk/index.html [Accessed 21-Jan-2012.
- Individual institutions are required to subscribe to the service.
- C. Tenopir, D. W. King, S. Edwards, and L. Wu, "Electronic journals and changes in scholarly article seeking and reading patterns," Aslib Proceedings, vol. 61, no. 1, pp. 5-32, 2009.
- The Pistoia Alliance, "SESL," 2012. [Online. Available: http://web.archive.org/web/20120712011655/http://www.pistoiaalliance.org... [Accessed: 21-Jan-2012.
- £416,168 x 144,000 = £59,927,040
- (31 minutes manual reading)/(5 minutes to read summary)
- M. Hucka et al., "Evolving a Lingua Franca and Associated Software Infrastructure for Computational Systems Biology - the Systems Biology Markup Language (SBML) Project.," Systems Biology, vol. 1, no. 1, pp. 41-53, 2004.
- J.-D. Kim, T. Ohta, K. Oda, and J. Tsujii, "From Text To Pathway: Corpus Annotation for Knowledge Acquisition From Biomedical Literature," Proceedings of The 6th Asia-Pacific Bioinformatics Conference, vol. 11, no. 1, pp. 165-175, 2008.
- E. Donnard et al., "Preimplantation development regulatory pathway construction through a text-mining approach," BMC Genomics, vol. 12, no. 4, p. S3, 2011.
- EMBL-EBI, "ChEBI." [Online. Available: http://www.ebi.ac.uk/chebi [Accessed: 12-Feb-2012.
- ChEBI has five curators.
- "Peer Review Workshop on the Value and benefits of text Mining to UKFHE, 10/02/12." 2012.
- B. Alex et al., "Assisted curation: does text mining really help?," Pacific Symposium on Biocomputing. Pacific Symposium on Biocomputing, vol. 567, pp. 556-67, Jan. 2008.
- It should be noted that the Alex, Grover et al [77] was a relatively small study; however, it is indicative of the types of efficiency savings that could be accrued.
- B. Alex et al., "Assisted curation: does text mining really help?," Pacific Symposium on Biocomputing. Pacific Symposium on Biocomputing, vol. 567, pp. 556-67, Jan. 2008.
- B. Alex et al., "Assisted curation: does text mining really help?," Pacific Symposium on Biocomputing. Pacific Symposium on Biocomputing, vol. 567, pp. 556-67, Jan. 2008.
- Alex, Grover at al [77] figures also include figures for text mining enhanced curation without curator assistance. These are omitted here for clarity as the curator assisted curation was the most efficient. See Alex, Grover at al [77] for full details of the experiment and their findings.
- Based on a curator as a non-academic professional with average salary of £36,040 (mid of salary scale £30,747 – £41,333) and 220 working days pa, 7.5 hours per day.
- "Mendeley," Mendeley. [Online. Available: http://www.mendeley.com [Accessed: 30-Nov-2011.
- "Start-Up of the Year" at the 2009 Plugg Conference; "Best Social Innovation Which Benefits Society" at the 2009 TechCrunch Europe Awards; "Start-Up Most Likely To Change The World For The Better" at the 2010 Guardian Activate Summit; "Best Education Start-Up" at the 2011 Telegraph 100; Microsoft/Sunday Times "Tech Track 100" Award 2011
- Not all publishers allow Mendeley to provide two page abstracts of their articles.
- Negotiations are underway with several others
- Mendeley, "Announcing the Mendeley Institutional Edition – powered by Swets," 2011. [Online. Available: http://web.archive.org/web/20120206062735/http://www.mendeley.com/blog/a... [Accessed: 14-Jun-2015.
- Mendeley, "Winners of the first Binary Battle Apps for Science Contest," 2011. [Online. Available: http://web.archive.org/web/20120211000447/http://www.mendeley.com/blog/d... [Accessed: 2015.
- I. Hargreaves, "Digital Opportunity: A Review of Intellectual Property and Growth," 2011.
- From the consultations it appeared that there is a larger body of 'text mining researchers' focused on the development of, and experimentation with, text mining tools, with a much smaller body of 'researchers using text mining' (ieusing text mining simply as another tool). Much of the UKFHE text mining tool development has focused on tools for biomedical uses – possibly, in part at least, as a natural result of the legal restrictions on material that can be text mined – since UK PubMed Central, as an Open Access repository, is one of the few current sources of material that can be legally text mined without seeking additional explicit permission.
- D. Delen and M. D. Crossland, "Seeding the survey and analysis of research literature with text mining," Expert Systems with Applications, vol. 34, no. 3, pp. 1707-1720, Apr. 2008.
- N. Harmston, W. Filsell, and M. P. H. Stumpf, "What the papers say: text mining for genomics and systems biology.," Human genomics, vol. 5, no. 1, pp. 17-29, Oct. 2010.
- U. Kelly, D. McLellan, and I. McNicoll, "The impact of universities on the UK economy," Journal of Policy Research in Tourism Leisure and Events, pp. 1-28, 2009.
- Comparable figures are not available for the FE sector across the UK, although a 2007 study of FE in England showed full-time equivalent employment of over 170,000 people and turnover of nearly £7bn
- Higher Education Statistics Agency, "Staff in Higher Education Institutions 2009/10," 2011.
- The 'headcount' equivalent was 181,595 people.
- We are basing our calculations on UK HESA data which gives a more conservative figure for the cost of researcher time than that used by CEPA. We are using Research Council norms for number of working hours per year (1,650 hours).
- This equates to 33 hours per full time equivalent academic per year (220 days and 44 working weeks per year).
- CIBER, "EVALUATING THE USAGE E-JOURNALS IN THE UK UPON BREADTH OF CITATION??," no. November. pp. 1-27, 2008.
- In collaboration with the Publishing Research Consortium, the Society of College, National and University Libraries (SCONUL) and Research Libraries UK (RLUK).
- Cambridge Economic Policy Associates, "Activities, costs and funding flows in the scholarly communications system in the UK Report commissioned by the Research Information Network ( RIN ) Full Report," 2008.
- It excluded other forms of scholarly outputs e.g. monographs, conference proceedings etc.
- Cambridge Economic Policy Associates, "Activities, costs and funding flows in the scholarly communications system in the UK: Summary Report."
- Cambridge Economic Policy Associates, "Activities, costs and funding flows in the scholarly communications system?: Key findings," 2008.
- C. Tenopir, D. W. King, S. Edwards, and L. Wu, "Electronic journals and changes in scholarly article seeking and reading patterns," Aslib Proceedings, vol. 61, no. 1, pp. 5-32, 2009.
- Of these 280 articles, 204 are unique.
- C. Tenopir, D. W. King, S. Edwards, and L. Wu, "Electronic journals and changes in scholarly article seeking and reading patterns," Aslib Proceedings, vol. 61, no. 1, pp. 5-32, 2009.
- Department for Business Innovation and Skills, "Innovation and Research Strategy for Growth," 2011.
- Further, a search for literature on the economic impact of copyright, market failure and related issues revealed very little hard or empirical evidence on the impact of copyright or on how copyright supported innovation – this evidence gap which was also noted in the BIS economic report
- European Commission, "INNOVATION UNION SCOREBOARD 2010," 2011.
- K. Allman et al., "Measuring Wider Framework Conditions for successful innovation: A system's review of UK and international innovation data," 2011.
- N. Miles, C. Wilkinson, J. Edler, M. Bleda, P. Simmonds, and J. Clark, "The wider conditions for innovation in the UK: How the UK compares to leading innovation nations," 2009.
- Higher Education Statistics Agency, "Finances of Higher Education Institutes 2009-2010." 2011.
- Department for Business Innovation and Skills, "Innovation and Research Strategy for Growth," 2011.
- Department for Business Innovation and Skills, "Innovation and Research Strategy for Growth," 2011.
- Department for Business Innovation and Skills, "Innovation and Research Strategy for Growth," 2011.
- H. Chesbrough, Open Innovation: The New Imperative for Creating and Profiting from Technology . Boston: Harvard Business School Press, 2003.
- A. L. Porter and N. C. Newman, "Mining external R&D," Technovation, vol. 31, no. 4, pp. 171-176, Apr. 2011.
- A. L. Porter and N. C. Newman, "Mining external R&D," Technovation, vol. 31, no. 4, pp. 171-176, Apr. 2011.
- Department for Business Innovation and Skills, "BIS Economics Paper No.15 Innovation and Research Strategy for Growth," no. 15. 2011.
- Department for Business Innovation and Skills, "BIS Economics Paper No.15 Innovation and Research Strategy for Growth," no. 15. 2011.
- E. Smit and M. Van der Graaf, "Journal article mining," 2011. Available: http://web.archive.org/web/20140102230201/http://publishingresearch.net/ [Accessed 13-Mar-2012
- Further, a search for literature on the economic impact of copyright, market failure and related issues revealed very little hard or empirical evidence on the impact of copyright or on how copyright supported innovation – this evidence gap which was also noted in the BIS economic report
- Welfare economics is a 'branch of economics that focuses on the optimal allocation of resources and goods and how this affects social welfare. Welfare economics analyses the total good or welfare that is achieved at a current state as well as how it is distributed'
- It is beyond the resources and the scope of this study to discuss the economic theory and its practical application in detail
- HM Treasury, The Green Book: Appraisal and Evaluation in Central Government, no. July. 2011, pp. 57-58.
- The 'Green Book' is a best practice guide in project and programme appraisal for government central departments and executive agencies. It draws on fundamental economic theory to give recommendations and guidance on economic, financial, social and environmental assessments.
- This is about the most effective use of resources to get the best overall outcome for society as a whole. An economy could be 'economically efficient' and still have disparities of wealth between citizens. It could mean for example that the rich get richer but as long as this does not mean that the poor get poorer this would be economically efficient.
- M. E. Porter, "Location, Competition, and Economic Development: Local Clusters in a Global Economy," Economic Development Quarterly, vol. 14, no. 1, pp. 15-34, Feb. 2000.
- M. Delgado, M. E. Porter, and S. Stern, "Clusters and entrepreneurship," Journal of Economic Geography, vol. 10, no. 4, pp. 495-518, May 2010.
- Knowledge spillovers are also part of theories of innovation
- E. Smit and M. Van der Graaf, "Journal article mining," 2011. Available: http://web.archive.org/web/20140102230201/http://publishingresearch.net/ [Accessed 13-Mar-2012
- The recent study into Journal Article Mining [8] indicated that 70% of the publishers surveyed required information from prospective users about the 'intent and purpose' of the mining request so that they could decide whether or not to grant permission.
- I. Hargreaves, "Digital Opportunity: A Review of Intellectual Property and Growth," 2011.
- British Library, "Response from the British Library to the Independent Review of Intellectual Property and Growth .," 2011.
- E. Smit and M. Van der Graaf, "Journal article mining," 2011. Available: http://web.archive.org/web/20140102230201/http://publishingresearch.net/ [Accessed 13-Mar-2012
- Department for Business Innovation and Skills, "BIS Economics Paper No.15 Innovation and Research Strategy for Growth," no. 15. 2011.
- R. Corrigan and M. Rogers, "The Economics of Copyright," English, vol. 6, no. 3, pp. 153-174, 2005.
- M. Rogers, J. Tomalin, and R. Corrigan, "The economic impact of consumer copyright exceptions?: A literature review."
- M. Ware and M. Mabe, "The stm report: An overview of scientific and scholarly journal publishing," 2009.
- Cambridge Economic Policy Associates, "Activities, costs and funding flows in the scholarly communications system in the UK Report commissioned by the Research Information Network ( RIN ) Full Report," 2008.
- The 'Innovation and Research Strategy for Growth' is BIS economics paper No. 15 http://bis.gov.uk/innovatingforgrowth
- Department for Business Innovation and Skills, "Innovation and Research Strategy for Growth," 2011.
- Department for Business Innovation and Skills, "Innovation and Research Strategy for Growth," 2011.
- Pricewaterhouse Coopers, "An economic analysis of copyright, secondary copyright and collective licensing," 2011.
- Cambridge Economic Policy Associates, "Activities, costs and funding flows in the scholarly communications system in the UK Report commissioned by the Research Information Network ( RIN ) Full Report," 2008.
About the authors

