Code of practice for learning analytics
Setting out the responsibilities of educational institutions to ensure that learning analytics is carried out responsibly, appropriately and effectively.
Introduction
Learning analytics uses data about students and their activities to help institutions understand and improve educational processes, and provide better support to learners.
Learning analytics should be used for the benefit of students. This might be to assist them individually or through using aggregated and anonymised data to help other students. Learning analytics might also be used to improve the educational experience more generally. It is distinct from assessment, and should be used for formative rather than summative purposes.
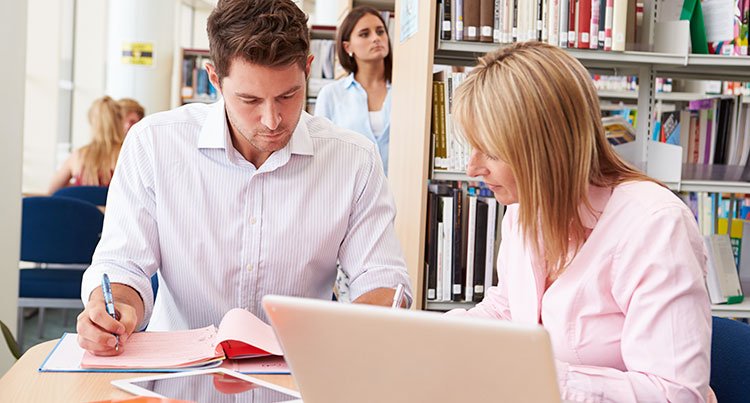
The effective use of learning analytics will initially involve the deployment of new systems along with changes to institutional policies and processes. New data may be collected about individuals and their learning activities. The data will be analysed and interventions may take place as a result. This presents opportunities for positive engagements and impacts on learning, as well as misunderstandings, misuse of data and adverse impacts on students.
Complete transparency and clear institutional policies are essential
Complete transparency and clear institutional policies are therefore essential regarding the purposes of learning analytics, the data collected, the processes involved, and how they will be used to enhance the educational experience.
This code of practice aims to set out the responsibilities of educational institutions to ensure that learning analytics is carried out responsibly, appropriately and effectively, addressing the key legal, ethical and logistical issues which are likely to arise.
Educational institutions in the UK already have information management practices and procedures in place and have extensive experience of handling sensitive and personal data in accordance with the Data Protection Act 2018. They must now also comply with the UK General Data Protection Regulation (GDPR).
By transferring and adapting this expertise to regulate the processing of data for learning analytics, institutions should establish the practices and procedures necessary to process the data of individuals lawfully and fairly.
Responsibility
Institutions must decide who has overall responsibility for the legal, ethical and effective use of learning analytics. They should allocate specific responsibility within the institution for:
- The collection of data to be used for learning analytics
- The anonymisation of the data where appropriate
- The analytics processes to be performed on the data, and their purposes
- The interventions to be carried out
- The retention and stewardship of data used for and generated by learning analytics
Student representatives and key staff groups at institutions should be consulted about the objectives, design, development, roll-out and monitoring of learning analytics.
Transparency and consent
Institutions will define the objectives for the use of learning analytics, what data is necessary to achieve these objectives, and what is out of scope. The data sources, the purposes of the analytics, the metrics used, who has access to the analytics, the boundaries around usage and how to interpret the data must be explained clearly to staff and students.
Institutions should also clearly describe the processes involved in producing the analytics to students and staff or make the algorithms transparent to them.
Institutions should clearly describe the processes involved in producing the analytics to students and staff
Student consent is required for the collection and use of "special category data", such as ethnicity, as defined by GDPR.
Students will also normally be asked for their consent for personal interventions to be taken based on the learning analytics. This will usually be done at the point where a specific intervention is proposed. However, there may be legal, safeguarding or other circumstances where students are not permitted to opt out of such interventions. If so these must be clearly stated and justified.
New learning analytics projects may not be covered by the institution’s existing arrangements. Collection and use of data for these may require further measures, such as data protection impact assessments and obtaining additional consent.
Options for granting consent must be clear and meaningful, explaining the expected consequences of granting or withholding consent. Students should be able easily to amend their decisions subsequently.
Privacy
Access to student data and analytics should be restricted to those identified by the institution as having a legitimate need to view them.
Where data is to be used anonymously particular care must be taken by institutions to avoid:
- Identification of individuals from metadata
- Re-identification of individuals by aggregating multiple data sources
The use of “special category data” for the purposes of learning analytics requires additional safeguards. Circumstances where data and analytics could be shared externally - eg requests from educational authorities, security agencies or employers - will be made explicit to staff and students, and may require additional consent.
The use of “special category data” for the purposes of learning analytics requires additional safeguards
Institutions should ensure that student data is protected when third parties are contracted to store data or carry out learning analytics on it.
Institutions may have a legal obligation to intervene, and hence override some privacy restrictions, where data or analytics reveal that a student is at risk. Such circumstances should be clearly specified.
Validity
It is vital that institutions monitor the quality, robustness and validity of their data and analytics processes in order to develop and maintain confidence in learning analytics and ensure it is used to the benefit of students. Institutions should ensure that:
- Inaccuracies in the data are understood and minimised
- The implications of incomplete datasets are understood
- The optimum range of data sources is selected
- Spurious correlations are avoided
All algorithms and metrics used for predictive analytics or interventions should be understood, validated, reviewed and improved as appropriate by qualified staff.
Data and analytics may be valid but should also be useful and appropriate; learning analytics should be seen in its wider context and combined with other data and approaches as appropriate.
Access
Students should be able to access all learning analytics performed on their data in meaningful, accessible formats, and to obtain copies of this data in a portable digital format. Students have a legal right under the GDPR to be able to correct inaccurate personal data held about themselves.
Students have a legal right to be able to correct inaccurate personal data held about themselves
They should normally also be able to view the metrics and labels attached to them. If an institution considers that the analytics may have a harmful impact on the student’s academic progress or wellbeing it may withhold the analytics from the student, subject to clearly defined and explained policies. However, the student must be shown the data about them if they ask to see it.
Enabling positive interventions
Institutions should specify under which circumstances they believe they should intervene when analytics suggest that a student could benefit from additional support. This may include advising students that they should not continue on a particular pathway. Students may also have obligations to act on the analytics presented to them – if so these should be clearly set out and communicated to the students.
The type and nature of interventions, and who is responsible for carrying them out, should be clearly specified. Some may require human rather than digital intermediation. Predictions and interventions will normally be recorded, and be auditable, and their appropriateness and effectiveness reviewed.
The impact of interventions on staff roles, training requirements and workload should be considered; addressing this requires support from senior management. Institutions should also be clear about the priority given to learning analytics in relation to other requirements.
Institutions should decide how to allocate resources for learning analytics appropriately for learners with different requirements and ensure that diverse groups and individuals are treated equitably.
Minimising adverse impacts
Institutions must recognise that analytics can never give a complete picture of an individual’s learning and may sometimes ignore personal circumstances.
Institutions should take steps to ensure that trends, norms, categorisation or any labelling of students do not bias staff, student or institutional perceptions and behaviours towards them, reinforce discriminatory attitudes or increase social power differentials.
Ensure that trends, norms, categorisation or any labelling of students do not bias staff, student or institutional perceptions and behaviours towards them
Analytics systems and interventions should be carefully designed and regularly reviewed to ensure that:
- Students maintain appropriate levels of autonomy in decision making relating to their learning, using learning analytics where appropriate to help inform their decisions
- Opportunities for “gaming the system” or any benefit to the student from doing so are minimised
- Knowledge that their activity is being monitored does not lead to non-participation by students or other negative impacts on their academic progress or wellbeing
- Adverse impacts as a result of giving students and staff information about the students’ performance or likelihood of success are minimised
- Staff have a working understanding of legal, ethical and unethical practice
Stewardship of data
Data for learning analytics must comply with existing institutional data policies and the GDPR and, in particular, be:
- Kept to the minimum necessary to deliver the purposes of the analytics reliably
- Processed in the UK, European Economic Area or, if elsewhere, only in accordance with the GDPR
- Retained only for appropriate and clearly defined periods
On request by students any personal data used for or generated by learning analytics should be destroyed or anonymised, with the exception of certain, clearly specified data fields required for educational or statutory purposes such as grades.
Published under the CC BY 4.0 license. Please cite as Jisc (2015): Code of practice for learning analytics.